This article introduces the paper "Defect reduction using Lean Six Sigma and DMAIC," providing a detailed overview of its methodology, findings, and implications for the die-casting industry.
1. Overview
- Title: Defect reduction using Lean Six Sigma and DMAIC
- Authors: Condé, G.C.P., Oprime, P.C., Pimenta, M.L., Sordan, J.L., Bueno, C.R.
- Year: August 2022
- Journal/Conference: Proceedings of the 5th ICQEM Conference, University of Minho, Portugal, 2022
- Keywords:
- Defect reduction
- Lean Six Sigma
- DMAIC
2. Research Background
- Social/Academic Context of the Research Topic:
- Competitive pressures force companies to seek solutions to eliminate wastes while improving product quality.
- Lean Six Sigma (LSS) has been considered one of the most effective approaches for business transformation.
- The auto parts sector has serious efficiency problems caused by high waste rates and unnecessary operations.
- Despite some applications of LSS and DMAIC in the automotive sector, these methodologies are still poorly applied in auto parts manufacturers according to literature.
- Limitations of Existing Research:
- While LSS and DMAIC literature contains many descriptions of practical applications, there is a need for detailed descriptions of the entire process used in structured improvement exercises within the auto parts manufacturing context.
- Necessity of Research:
- To present a detailed description of an empirical case study where Lean Six Sigma and DMAIC methodologies are applied to reduce defects in an auto parts manufacturer.
- To provide a reference for those intending to use similar improvement methodologies for defect reduction in auto parts manufacturing.
3. Research Objectives and Research Questions
- Research Objective:
- To present an empirical case study of defect reduction in an auto parts manufacturer using Lean Six Sigma and DMAIC methodologies.
- To describe in detail the entire process used in a structured improvement exercise.
- Core Research Questions:
- How can Lean Six Sigma and DMAIC methodologies be effectively applied to reduce defects in die-casting and machining processes within an auto parts manufacturing company?
- What are the key variables influencing defect rates in these processes?
- What solutions can be implemented to sustainably reduce defect incidence and improve sigma levels?
- Research Hypothesis:
- The application of DMAIC methodology within a Lean Six Sigma framework can lead to a significant and sustainable reduction in defects in the die-casting and machining processes of auto parts manufacturing.
4. Research Methodology
- Research Design:
- Empirical single longitudinal case study.
- DMAIC methodology (Define, Measure, Analyse, Improve, Control) was followed.
- Data Collection Methods:
- Semi-structured interviews conducted in the DMAIC steps sequence.
- Document analysis.
- Direct field observations.
- Analysis Methods:
- Statistical analysis using Minitab.
- Design of experiments (factorial experiments).
- Hypothesis testing.
- Cause and Effect Matrix.
- Measurement System Analysis (MSA).
- Process Capability Analysis.
- Research Subject and Scope:
- A manufacturing company in Brazil producing die-casting and machined aluminum auto parts for major vehicle manufacturers.
- The study focused on defect reduction in the die-casting and machining processes for a specific auto part: Rearview Housing Support (RHS).
5. Key Research Findings
- Core Research Findings:
- The analysis pointed out the main defects in die casting and machining phases.
- Die Casting: "Mold temperature, metal temperature and second stage injection speed influenced the amount of defective die casting parts."
- Machining: "On the other hand, the incidence of defects in machining process was affected by the fixation method."
- "Solutions implemented reduced the defect incidence from a chronically high level to an acceptable one."
- "The sigma level rose from 3.4 σ to 4σ sustainably."
- Statistical/Qualitative Analysis Results:
- Define Phase: "Two control charts were elaborated: p-chart for rejection rate of die-casting parts (Figure 1), and p-chart for rejection rate of machining parts (Figure 2)." These charts showed processes with special causes and high defect rates. Baseline sigma level was calculated as "3,4 sigmas".
- Measure Phase: "Project Team prepared a SIPOC (Suppliers, Input, Process, Output, Customers) matrix (Figure 3)." Detailed variable analysis identified 30 possible variables impacting defect fraction (Table 3). "Cause and Effect Matrix" prioritized seven key variables (Table 4 and 5). "Measurement System Analysis (MSA)" confirmed acceptable measurement system ("all Kappa are above 0,7" in Table 7).
- Analyse Phase: "Ishikawa Diagrams" (Figure 7 and Figure 8) were used for root cause analysis. "A series of factorials experiments" (Figure 9, 10, 13, 14) identified significant variables:
- Die-casting: Mold Temperature (x1), Metal Temperature (x2), and 2nd phase injection speed (x3). Optimal combination found in Figure 12: "Mold Temperature in the level = 220° C, Metal Temperature in the level = 700° C, and injection speed (Phase 2) in the level 3 meters per second".
- Machining: Fixation method (x5). Optimal method found in Figure 16: "type II (alternative) fixation method - x5, named “Torres”".
- Improve Phase: "Decision Matrix" (Table 10) ranked and selected four solutions for implementation: "Thermal oil using, New Machining Fixation Method, Improve maintenance of foundry components, Alternative tool holder type."
- Control Phase: "Hypothesis test" (Figure 18 and Figure 19) confirmed significant reduction in defect rates for both die-casting and machining processes ("P-Value = 0,000"). "Process Capability Analysis" (Figure 21 and Figure 22) showed improved and sustained process performance, reaching "4σ" sigma level (Figure 20).
- Data Interpretation:
- Statistical analysis and experimental results demonstrated the effectiveness of DMAIC methodology in identifying and addressing root causes of defects.
- Optimized parameters and implemented solutions led to a significant and sustainable reduction in defect rates and improved process sigma level.
- Figure Name List:
- Figure 1 - p-chart for rejection rate of die-casting parts.
- Figure 2 - p-chart for rejection rate of machining parts.
- Figure 3 - SIPOC / Die-casting and machining housing support manufacturing process.
- Figure 4 - Part regions.
- Figure 5 - Final Process Yield calculation – before improvements.
- Figure 6 - Pareto Chart – Main reasons for rejecting parts.
- Figure 7 - Root cause analysis for selected variable x1 (mold temperature).
- Figure 8 - Root cause analysis for selected variable x7 (cutting Tool Type - Thread).
- Figure 9 - Pareto Chart of the Effects of the first experimental running – Die-casting.
- Figure 10 - Pareto Chart of the Effects of the second experimental running – Die casting.
- Figure 11 - Interaction on second experimental running – Die Casting.
- Figure 12 - Best combination of variables found during second experimental running – Die casting.
- Figure 13 - Pareto Chart of the Effects of the first experimental running – machining.
- Figure 14 - Pareto Chart of the Effects of the second experimental running – machining.
- Figure 15 - Interaction on second experimental running – machining.
- Figure 16 - Best combination of variables found during second experimental running – die casting.
- Figure 17 - Project Team meetings.
- Figure 18 - Hypothesis Test for die-casting improvements.
- Figure 19 - Hypothesis Test for machining improvements.
- Figure 20 - Final Process Yield calculation - improved processes.
- Figure 21 - Periodic process Capability Analysis – Die casting process.
- Figure 22 - Periodic process Capability Analysis – machining.
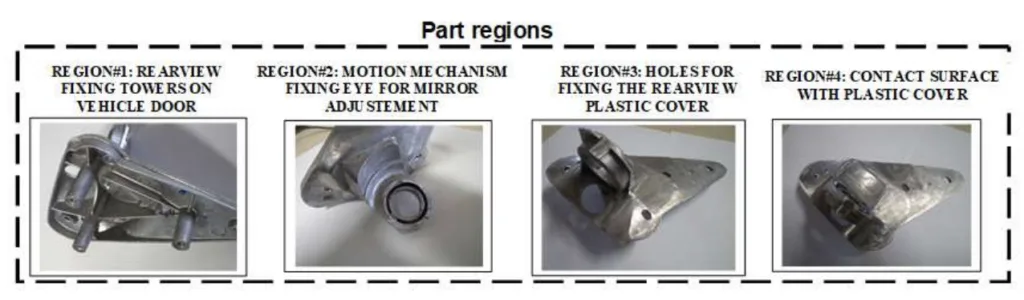
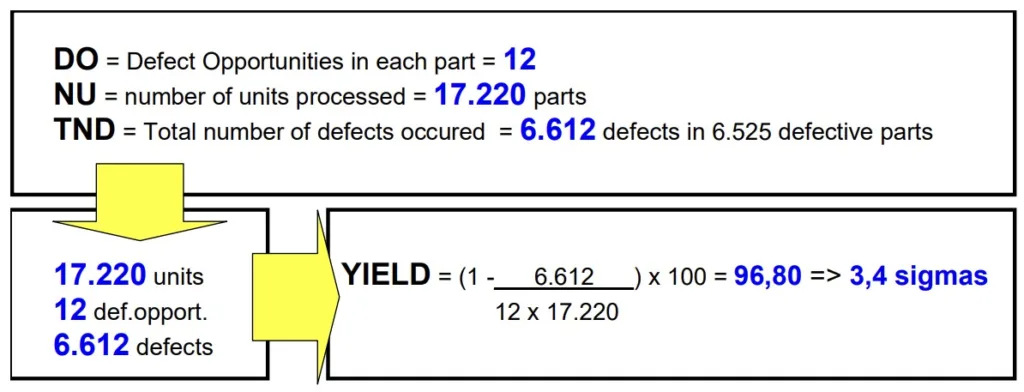
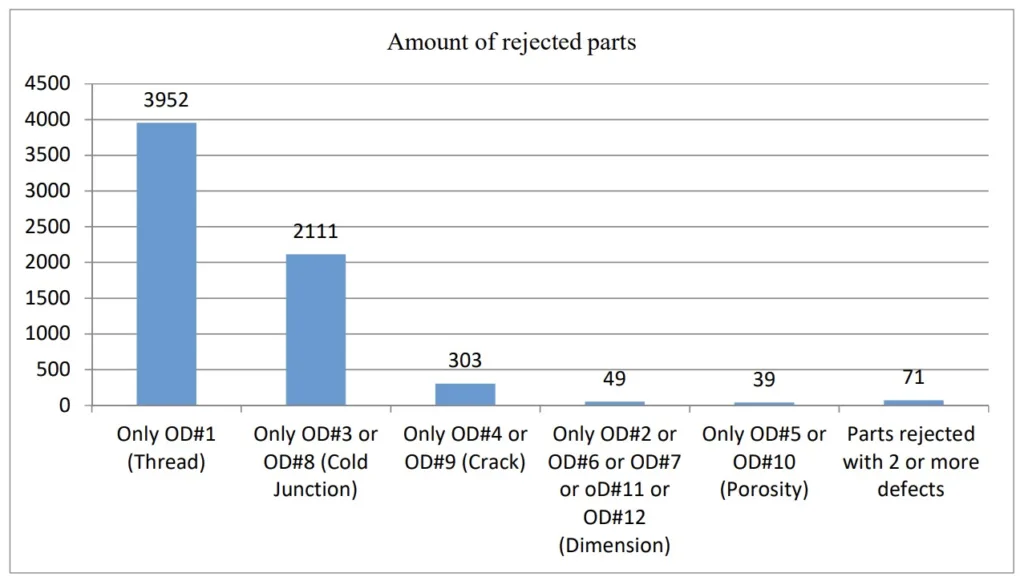
6. Conclusion and Discussion
- Summary of Main Results:
- The project successfully applied DMAIC methodology to reduce defects in die-casting and machining processes.
- Key variables influencing defects were identified and optimized.
- Implemented solutions led to a sustainable increase in sigma level from "3.4 σ to 4σ".
- Project goals of reducing reject rates to less than "7% in die-casting process" and less than "1% in machining process" were achieved.
- Academic Significance of the Research:
- Provides a detailed empirical case study demonstrating the effective application of Lean Six Sigma and DMAIC in auto parts manufacturing.
- Contributes to the literature by illustrating the step-by-step process of DMAIC implementation, including the use of specific tools and techniques.
- Practical Implications:
- This paper can be used for those who intend to use the same type of improvement methodologies.
- This study can be used as a reference for managers and engineers in the auto parts industry seeking to implement Lean Six Sigma projects for defect reduction.
- The detailed methodology and identified key variables provide practical guidance for similar improvement initiatives.
- The successful case study demonstrates the potential of DMAIC to achieve significant and sustainable improvements in manufacturing processes.
- Limitations of the Research:
- "The study are limited to a single case study, without intention of generalizing the results to other types of industries."
7. Future Research
- Directions for Future Research:
- Further research could explore the generalizability of these findings to other types of industries and manufacturing processes.
- Investigating the application of LSS and DMAIC in other areas of auto parts manufacturing beyond defect reduction could be beneficial.
- Areas for Further Exploration:
- Exploring the long-term sustainability of the achieved improvements and the factors influencing it.
- Investigating the impact of organizational culture and employee engagement on the success of LSS and DMAIC implementation in auto parts manufacturing.
8. References
- Antony, J. (2015), “Challenges in the deployment of LSS in the higher education sector: Viewpoints from leading academics and practitioners”, International Journal of Productivity and Performance Management.
- Antony, J., Antony, F. J., Kumar, M. and Cho, B. R. (2007). “Six sigma in service organisations: Benefits, challenges and difficulties, common myths, empirical observations and success factors", International journal of quality & reliability management, Vol. 24 No. 3, pp. 294-311.
- Bass, I., and Lawton, B. (2009). Lean six sigma using SigmaXL and Minitab. McGraw Hill Professional.
- Bayou, M. E. and De Korvin, A. (2008). “Measuring the leanness of manufacturing systems—a case study of Ford Motor Company and General Motors”, Journal of Engineering and Technology Management, Vol. 25 No 4, pp. 287-304.
- Chaurasia, B., Garg, D., & Agarwal, A. (2019), “Lean Six Sigma approach: a strategy to enhance performance of first through time and scrap reduction in an automotive industry”, International Journal of Business Excellence, Vol. 17, No. 1, pp. 42-57.
- Costa, L. B. M., Godinho Filho, M., Fredendall, L. D. and Ganga, G. M. D. (2021), “Lean six sigma in the food industry: Construct development and measurement validation”, International Journal of Production Economics, Vol. 231, pp. 107843.
- Chua, R. C. H. and DeFeo, J. A. (2006), Juran's quality planning and analysis: For enterprise quality. Tata McGraw-Hill Education.
- Daniyan, I., Adeodu, A., Mpofu, K., Maladzhi, R. and Katumba, M. G. K. Κ. (2022), “Application of lean Six Sigma methodology using DMAIC approach for the improvement of bogie assembly process in the railcar industry”, Heliyon, e09043.
- Doolen, T. L. and Hacker, M. E. (2005), “A review of lean assessment in organizations: an exploratory study of lean practices by electronics manufacturers”, Journal of Manufacturing systems, Vol. 24 No. 1, pp. 55-67.
- George, M. L., Maxey, J., Rowlands, D. T., & Upton, M. (2004). Lean six sigma pocket toolbook. McGraw-Hill Professional Publishing.
- Gupta, S., Modgil, S. and Gunasekaran, A. (2020), “Big data in lean six sigma: a review and further research directions”, International Journal of Production Research, Vol. 58 No. 3, pp. 947-969.
- International Organization for Standardization. ISO 13.053-1: Quantitative methods in process improvement – Six Sigma Part 1: DMAIC methodology. Genebra: ISO, 2011.
- Kahraman, C., and Büyüközkan, G. (2008), “A Combined Fuzzy AHP and Fuzzy Goal Programming Approach for Effective Six-Sigma Project Selection”, Journal of Multiple-Valued Logic & Soft Computing, Vol. 14 No. 6.
- Karlsson, C. and Åhlström, P. (1996). “Assessing changes towards lean production", International Journal of Operations & Production Management.
- Kwak, Y. H., and F. T. Anbari. (2006), “Benefits, Obstacles, and Future of Six Sigma Approach”, Technovation, Vol. 26 No. 5–6, pp. 708–715.
- Mancilla-Escobar, J., Maccasi-Jaurequi, I., Raymundo-Ibañez, C. and Perez, M. (2020, March). Layout Lean model of production management based on change management to improve efficiency in the production of packaging in auto parts sector SMEs. In IOP Conference Series: Materials Science and Engineering (Vol. 796, No. 1, p. 012014). IOP Publishing.
- McLachlin, R. (1997), “Management initiatives and just-in-time manufacturing”, Journal of Operations management, Vol. 15 No. 4, pp. 271-292.
- Mishra, Y., Sharma, M. K., Yadav, V., Meena, M. L. and Dangayach, G. S. (2021), “Lean Six Sigma implementation in an Indian manufacturing organisation: a case study”, International Journal of Six Sigma and Competitive Advantage, Vol. 13 No. 1-3, pp. 76-100.
- Panizzolo, R. (1998), “Applying the lessons learned from 27 lean manufacturers.: The relevance of relationships management”, International journal of production economics, Vol. 55 No. 3, pp. 223-240.
- Pinheiro, T. H., Scheller, A. C., & Miguel, P. A. C. (2013), “Integração do seis sigma com o lean production: uma análise por meio de múltiplos casos”, Revista Produção Online, Vol. 13 No. 4, pp. 1297-1324.
- PMBOK, G. (2008). Um guia do conjunto de conhecimentos em gerenciamento de projetos (guia pmbok®). Project Management Institute, Inc. EUA.
- Salah, S., Rahim, A. and Carretero, J. A. (2010), “The integration of Six Sigma and lean management", International Journal of Lean Six Sigma.
- Schroeder, R. G., Linderman, K., Liedtke C. and Choo, A.S. (2009), “Six Sigma: Definition and Underlying Theory.” Quality Control and Applied Statistics, Vol. 54 No. 5: pp. 441–445.
- Shah, R. and Ward, P. T. (2003), “Lean manufacturing: context, practice bundles, and performance", Journal of operations management, Vol. 21 No. 2, pp. 129-149.
- Shokri, A. (2019), “Reducing the scrap rate in manufacturing SMEs through lean six sigma methodology: An action research”, IEEE Engineering Management Review, Vol. 47 No. 3, pp. 104-117.
- Snee, R. D. (2004), “Six-Sigma: The Evolution of 100 Years of Business”, International Journal of Six Sigma and Competitive Advantage, Vol. 1 No. 1: pp. 4-20.
9. Copyright
- This material is based on the paper by [Giovanni Condé, Pedro Carlos Oprime, Pimenta, M.L., Sordan, J.L., Bueno, C.R.]: [Defect reduction using Lean Six Sigma and DMAIC].
- Paper Source: https://www.researchgate.net/publication/362389760
This material is a summary based on the above paper, and unauthorized use for commercial purposes is prohibited.
Copyright © 2025 CASTMAN. All rights reserved.