- SOCIETAL LCA
- Open access
- Published: 26 April 2024
- Cite this article
You have full access to this open access article
The International Journal of Life Cycle AssessmentAims and scopeSubmit manuscript
Social hotspots in the automotive industry’s aluminum value chains—combining primary and generic data
- 662 Accesses
- Explore all metrics
Abstract
Purpose
The automotive industry and its suppliers have been facing increasing challenges in the sustainable sourcing of raw materials on environmental and social levels. To identify social risks in the value chain of aluminum in the automotive sector, this study aimed to examine how a generic hotspot analysis based on S-LCA can be conducted and support the reporting according to new legislative requirements such as the German Act on Corporate Due Diligence Obligations in Supply Chains.
Methods
This study investigated the social risks in the supply chains of two selected aluminum components of an aluminum company using a generic social hotspot analysis based on the UNEP Guidelines for Social Life Cycle Assessment of Products and Organizations 2020. The study employed the generic PSILCA© database and different additional indicators for the assessment. The UNEP Guidelines 2020 used in combination with the associated methodological sheets were beneficial in structuring the procedure within the social hotspot analysis. They gave the inexperienced user a guide, which led to the results presented in this work.
Results and discussion
A high number of social hotspots in upstream processes outside Europe across the different investigated indicators for both supply chains assessed were detected. Most social risks were identified in Guinea, as well as in a European and an Asian Country in the stakeholder categories “Workers” and “Local community.” Especially, the alloy element production in the Asian Country and the bauxite mining in Guinea showed an above-average number of social hotspots. The implementation of the work was associated with an enormous effort in information collection about the supply chains to be investigated and depended on the direct suppliers’ willingness to contribute. The need for a safe and supporting environment for suppliers to enhance transparency was identified.
Conclusions
Combining generic and company data to conduct a social hotspot assessment (e.g., using PSILCA©) to meet legislative requirements proved to be a suitable approach for complex supply chains such as the automotive one. Despite this, limitations of the PSILCA© database and potential for improvement were acknowledged regarding industry-wide social assessments of supply chains. Additionally, the study serves as an application example of the UNEP Guidelines 2020 and the activity variables for science in practice. Further development and standardization of social indicators and activity variables for impact measurement were identified as a research priority.
Similar content being viewed by others
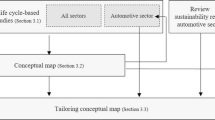
Analysis of the main elements affecting social LCA applications: challenges for the automotive sector
Article 29 July 2016
Assessment of Social Impacts Along the Value Chain of Automation Technology Components Using the LCWE Method
Chapter © 2019
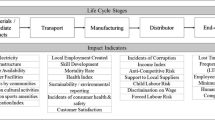
Social life cycle assessment in Indian steel sector: a case study
Article 22 December 2017
1 Introduction
In an increasingly complex economic world, the sustainability requirements for companies are growing among various stakeholders, customers, NGOs, and administrations. The German Act on Corporate Due Diligence Obligations in Supply Chains (Bundestag 2021) strengthens the need for cooperation in risk assessments, transparency, and taking actions in the worldwide supply chains regarding environmental risks and human rights as of 2023. The act provides regulations for due diligence obligations, responsibility for the supply chain, external reviews, and the protection of human rights. It addresses a company’s own business area, its direct contract partners, and indirect suppliers. Related to human rights, the focus is on child labor, forced labor, slavery, occupational health and safety, freedom of association, discrimination, fair salary, harmful soil change, pollution, unlawful seizure of land, and security forces. At present, the knowledge about the processes along the supply chains is often limited to the well-known in-house processes and direct suppliers. The consideration of social aspects in the entire value chain, which is possible by carrying out a social life cycle assessment (S-LCA), is not common practice yet.
Aluminum is an essential raw material for the automotive industry and was the focus of this study. Assessing the social risks of aluminum production considering the supply chain is crucial as it is linked to various social risks. Bauxite mining operations, for example, can be responsible for partial deforestation of primary rainforests in countries like Guinea, Ghana, and Brazil, causing the endangering of indigenous rights and local populations in these regions (Burger 2014; Vasters and Franken 2020; Fouche 2021). The mining operations can also degrade rivers and streams around the mining areas and mine dust from material handling operations, crushing, blasting, drilling, and haul roads can cause nuisance and aesthetics to the mine workers (Keita 2020). When processing bauxite to alumina in the Bayer process, red mud is generated as a waste product, leading to social and environmental issues due to its high alkalinity and the potential of tailing dam failure (Sushil and Batra 2008; Bruschi et al. 2021). Last, due to smelting operations in the Hall-Héroult process, heat stress can occur and inhalation hazards represent the most common mechanism for potentially injurious exposure in primary aluminum production. The symptoms of cough, wheezing, rhinitis, and the quantifiable presence of obstructive or restrictive lung disease are at the center of consideration in the aluminum industry (Martin and Larivière 2014; Wesdock and Arnold 2014).
Even though the other pillars of sustainability (environmental and economic aspects) were considered recently in studies from the aluminum sector (Luthin et al. 2021; Milovanoff et al. 2021; Schiessl et al. 2021), only one S-LCA of aluminum profiles for windows was found (Di Giorgio et al. 2022). The authors did not find any S-LCA study on the aluminum value chain in the automotive sector which was the motivation for this study.
This study tested the possibility of determining the social risks of a complex product and supply chain using a social hotspot analysis based on the UNEP Guidelines for Social Life Cycle Assessment of Products and Organizations 2020 (UNEP 2020). Social hotspots on the country and sector level of two advanced aluminum components in an electro-mobility product were assessed in cooperation with the company producing the components. One component consists of high purity primary aluminum and the other component consists of an aluminum alloy made entirely of secondary material. A social hotspot in this study was defined as a location or process in a life cycle in which social risks or social issues occur concerning the previously defined subcategories. Defined stakeholder categories, impact categories, and social indicators of the PSILCA© database V2 were used. PSILCA© was chosen as it is based on the structure of the Methodological Sheets for Sub-Categories in Social Life Cycle Assessment (UNEP 2013). The data was obtained from interviews with the direct company’s suppliers, literature research, the generic PSILCA© V2 database, and questionnaires specially developed for this work to query the entire aluminum supply chains with the help of the direct suppliers. The social hotspot analysis was intended to contribute to the practice-oriented application development of social risk assessment along the often very complex supply chains in the automotive industry related to customer requirements and legislation (Karlewski et al. 2019). It can help to concretize future process and system requirements around social sustainability in the supply chains. Moreover, the study showed the importance of using S-LCA as a supporting tool for addressing social due diligence according to the German Act on Corporate Due Diligence Obligations in Supply Chains (Bundestag 2021).
2 Aluminum
Aluminum is the most abundant metallic element in the earth’s crust and one of the most significant metals used in modern technologies (Kvande 2011). Due to its physical properties, aluminum can be used in a wide range of applications. With its lightweight and good processability, it is used in modern automotive products, in the aviation industry, or in further transportation systems (Menzie et al. 2010). Depending on its intended application, aluminum is formed into different semi-manufactured goods processed at the end of the value chain into finished goods. For every 4 to 6 kg of bauxite (ore), which is considered a critical raw material in the European Union (EU) and that comes with economic relevance as well as with environmental and social supply chain risks, approximately 2 kg of alumina and resulting of this 1 kg of primary aluminum is produced (Wesdock and Arnold 2014). The connected process steps can include adding alloying elements, casting the metal into shapes, rolling the metal into sheets, drawing the metal into wire, or extruding the metal to produce different shapes. Figure 1 indicates the worldwide karstic and lateritic bauxite deposits.
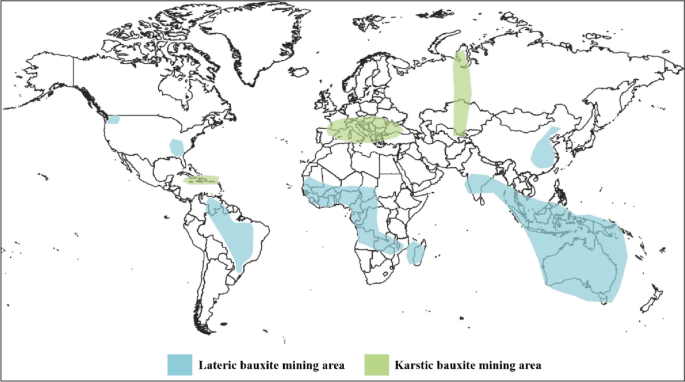
In addition to producing primary aluminum from aluminum oxide through electrolysis, secondary aluminum is produced by processing aluminum scrap and production wastes into usable secondary aluminum (Menzie et al. 2010; Mahinroosta and Allahverdi 2018). In Europe, the total use of secondary materials in aluminum production is over 50% (Luthin et al. 2021). The need for only 5% of the energy required for primary production is the most important benefit of aluminum recycling (Wallace 2011; Zimring 2016; Luthin et al. 2021).
3 Social life cycle assessment
Life cycle thinking is a concept for the identification of impacts of products along their supply chains and their whole life cycle. Life cycle assessment (LCA) was first developed as a standardized method for analyzing and measuring the environmental impact of products along their life cycle (ISO 2006). Based on this, S-LCA was developed to assess the potential positive and negative social and socio-economic impacts of products and services across their life cycle (Mancini et al. 2018; UNEP 2020). In 2009, the UNEP/SETAC Life Cycle Initiative published the first guidelines for S-LCA which were updated in 2020 (UNEP 2020).
S-LCA assesses the impacts generated on relevant stakeholders along a product’s life cycle. It includes the definition of scope and goal, a social life cycle inventory analysis, a social life cycle impact assessment (S-LCIA), and the interpretation of the results (UNEP 2020). The iterative approach allows the goal and scope to be adjusted and modified during implementation to accommodate changing conditions and newly added data (Werker et al. 2019). Based on the defined system boundaries, relevant data is collected in the social life cycle inventory process. Here, the material flows and further information on the investigated system, required for the following S-LCIA, are collected. During the S-LCIA, potential social risks and benefits are linked to the social life cycle inventory results and calculated using the methodological framework. Two kinds of impact assessments of S-LCA exist: the reference scale approach (Type I) and the impact pathway approach (Type II). The reference scale approach focuses on the social performance of activities in a product system based on reference points (e.g., sector average, targets, norms). The impact pathway approach focuses on the consequences and long-term implications of activities using causal or correlation-based relationships between the product system’s activities and resulting social impacts (UNEP 2020). In the final step of an S-LCA, the results are interpreted, and further measures and recommendations are derived (Werker et al. 2019; UNEP 2020).
Precise site-specific data of the different process locations along the value chains are required to apply S-LCA in the context of product assessments. A hotspot analysis can also be applied if there is no such information about the process sites available or if a more generic overview of social risks is required (Thies et al. 2019). Depending on the approach and social indicators considered, a social hotspot is defined as a specific region where positive, problematic, or risky situations can occur (UNEP 2020). Specifically, databases are used to assess country and sector-based social risks to analyze social hotspots at a generic level. The currently available social databases are the Social Hotspot Database (SHDB) and the Product Social Impact Life Cycle Assessment database (PSILCA©). The database used in this study was PSILCA© V2 published by GreenDelta Gmbh. It includes the stakeholders and subcategories identified in the old version of the Methodological Sheets (UNEP 2013).
Applications of the UNEP Guidelines for S-LCA or hotspot analyses have been steadily advanced since the first publication, and the methods have been applied in various sectors (UNEP 2020). The implementation areas range from the assessment of textile supply chains (Martin and Herlaar 2021) over complex supply chains of the Li-ion battery industry (Thies et al. 2019) to raw material or sector-specific studies (Mancini et al. 2018; Werker et al. 2019). Karlewski et al. (2019) noted in their paper on S-LCA in the automotive industry that S-LCA studies with company-specific data are also feasible in an industry with complex suppliers. According to Karlewski et al. (2019), the main difficulties and limitations in the automotive industry are the standardization of indicators, the lack of knowledge about applied processes in the supply chains, and the lack of experience in interpreting the S-LCA outputs.
4 Methodology
To identify social risks in the aluminum value chain, a social hotspot analysis based on the Guidelines for Social Life Cycle Assessment of Products and Organizations (UNEP 2020) was conducted for two different aluminum products. OpenLCA© and the social risk indicator database PSILCA© V2 from 2017 developed by GreenDelta GmbH were used in this study. The study was conducted in cooperation with the company producing the aluminum components (last supply chain actor within system boundaries) that provided relevant data and process information.
4.1 Case study
Together with the company, requirements were defined to identify two relevant and suitable aluminum products whose social risk assessment was most favorable for the company in terms of the importance of internal and external communication:
- 1.The products had to be part of an electromobility project because rising requirement pressure on questions of social aspects is expected from the automotive manufacturers in the future.
- 2.The products should preferably be in series production, or production starts in the future.
- 3.In the best case, an environmental LCA has already been completed to tackle a further dimension of sustainability with the social hotspot analysis.
- 4.The products and suppliers must be approved for the project by the respective category manager.
- 5.The supplier had to agree to participate in the project and to answer the supplier questionnaire.
Based on the requirements, the study focused on the rotor of an asynchrony electric motor as part of an OEM (original equipment manufacturer) drive system, which serves as a drive unit for the movement of an electric car. The rotor itself is the moving part of the electric motor. The fixed stator around the rotor generates a rotating magnetic flux in its copper windings and permanent magnets, closing via the rotor. A circumferential force is created in the rotor through the asynchronous rotation of the magnetic flux compared to the rotor, which causes the rotor to rotate. Within the rotor, two aluminum components with two different functions were analyzed, enabling in their interaction the function of the rotor and consequently of the drive unit. The first considered component, “High purity aluminum”, was the rotor cooling system’s die-casted aluminum around an iron squirrel cage. The high purity aluminum is cast around the iron squirrel cage through a die-casting process. The iron inside the cage was not examined in this work. The used aluminum has high purity and good conductivity, enabling the internal rotor cooling via convective heat discharge (Doerr et al. 2019). For one casting process, 2 kg of pure aluminum is needed, with 1 kg being used in the product, and 1 kg being production waste (intended to be added back to the die-casting process). Due to its high purity, the purchased aluminum ingots need to originate from primary aluminum. The second considered component, “Balancing disks”, consists of two aluminum alloy balancing disks on both ends of the rotor that prevent the rotor system from unbalancing while rotating. The weights of the balancing disks are approximately 0.2 kg. Both consist of secondary aluminum alloy. Secondary material is used in this product since the chemical composition is irrelevant to achieving the function and it is much more cost-effective than primary aluminum. Furthermore, the alloying elements in the secondary aluminum ensure better machinability in further processing (Cui and Roven 2010). After producing the rotor with its aluminum components in the company’s assembly line, the rotor is delivered to the OEM side for further production steps. In this phase of the project, each category is delivered by one individual supplier.
4.2 Data collection from suppliers
To not disclose or jeopardize business relationships, the selected suppliers were used anonymously in this study. They were referred to by the names “Supplier 1” for the category “Balancing disks” and “Supplier 2” for the category “High purity aluminum.” In cooperation with company experts in materials technology, a questionnaire was created based on the processes within the system boundaries of primary and secondary aluminum production to query information for the respective product, sub-suppliers’ location of production, and mass balance information.
To motivate the suppliers to participate, the first section explained the background and purpose of the study. In addition, an overview of the advantages the supplier could gain from answering the questionnaire for future legislation, such as the German Act on Corporate Due Diligence Obligations in Supply Chains (Bundestag 2021), was given. It was complemented by a disclaimer, which assured the supplier that the data was used anonymously. The second section included a glossary of terms used in the questionnaire to ensure the completion was facilitated. Furthermore, the supplier was shown examples from fictitious supply chains to prevent any queries that may arise. In the last section, the required information was collected from the supplier. In addition to general information on location and contact addresses, specific information on the internal manufacturing processes of the queried product and additional supply chain actors was requested. The query of the supplier network was mandatory at the country and sector level. This approach was chosen so that suppliers would see the collaboration as a confidence-building measure in the mutual business relationship and not reject the requests as overwhelming. The information from the questionnaire was used to supplement the supply chain outlined by literature research.
4.3 Functional unit and system boundaries
The functional units for the chosen products described earlier were “Balancing disks” with the function of preventing unbalancing of the rotor and the die-cast “High purity aluminum” with the function of magnetic field conduction and rotor cooling.
For reasons of confidentiality, several countries were anonymized according to the respective continent to ensure the company’s anonymity: European country (EC), Asian country (AC), African country (AfC), North America (NA), and South America (SA). Countries that do not reveal the identity of the company are named in the study.
Each of the two components, “High purity aluminum” and “Balancing disks,” have separate supply chains, which unite in the company’s production plants in EC3. For this reason, in addition to the fact that “High purity aluminum” involves high purity aluminum and the “Balancing disks” consist of a secondary aluminum alloy, two reference flows were used. All impacts were related to 1 t of the component “High purity aluminum” and 1 t of “Balancing disks”. The sourced materials were each assigned a monetary value per ton per process. Due to the confidentiality agreement with the suppliers, the values and prices determined are not presented in this paper.
A cradle-to-gate approach was used in the study. The system boundaries, as shown in Fig. 2, included all relevant manufacturing processes required to produce the two selected components and the rotor itself. The component “Balancing disks” is made of aluminum scrap from EC1 and EC2 with additions of the alloying elements silicon and magnesium from AC1 and copper scrap from AC2. The processes were divided into the final assembly of the balancing disks at the company’s plant in EC3, the chip removal process at the plant in EC1, the die casting production of the alloy bars at “Supplier 1” in AC2, and the casting of the alloy as aluminum ingots at the direct supplier of “Supplier 1.” The production process of magnesium and silicon alloying elements in AC1 was not included in the product system and only the country of origin was specified. For the scrap and alloying elements, information on the maximum use in the process was given in percent. After the foundry process, the secondary aluminum ingots are delivered to “Supplier 1.” The ingots are first melted and then pressed into the required shape by a die-casting process. After the die-casting process, the surface is treated. CNC machines remove the remaining production residues before leaving the plant in AC2 towards the company’s plant in EC1. The component “High purity aluminum” was produced entirely from primary aluminum. The primary aluminum route was composed of bauxite mining, further processing into alumina, and the subsequent electrolysis process, including the associated foundry processes. In the case of high purity aluminum, ingots were supplied by the trader “Supplier 2”. The electrolysis, respectively, the direct supplier of “Supplier 2” of the material, took place in EC4 through nine different alumina suppliers based in Europe and South America. The alumina producers received the bauxite from different mines, mostly in the same countries as their refiners. The high purity aluminum provided by “Supplier 2” was further processed at the company’s plant in EC3, in the aluminum die-casting process, and was assembled at the exact location as a component in the end product, the rotor of the electric motor.
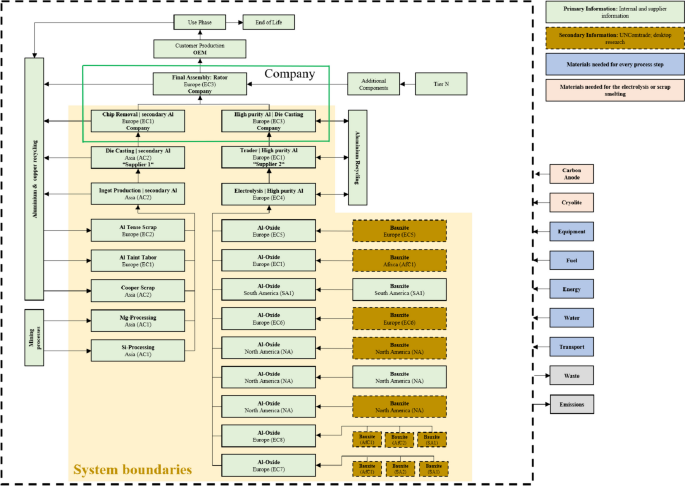
The main in- and outputs of the product system were defined as transport efforts between the locations, water and energy usage, waste production like red mud in the refinery process, emissions, and specified inputs for the two aluminum production routes (Liu and Wu 2012; Keita 2020). The specified inputs for the aluminum production were limited to the used cryolite and carbon anodes for the electrolysis process and the carbon anodes for the secondary aluminum production process (Bergsdal et al. 2004; Vasters and Franken 2020). Chemicals used in the refinery process, the fuels, and the equipment for the bauxite mining, respectively, for transport, and the scrap collection processes were identified as additional inputs in the system. Waste and emissions could be considered outputs of the system in addition to the aluminum products. The additives required for the respective process steps, such as chemicals and carbon anodes, energy flows, transport effort, and the waste produced, were not included due to the low information density from the suppliers and for reasons of limitation. Aluminum scrap collection was also not considered.
4.4 Stakeholder categories, subcategories, and indicators
The Methodological Sheets for Subcategories in Social Life Cycle Assessment (S-LCA) (UNEP 2021) were the starting point for selecting stakeholder categories, subcategories, and indicators for the social hotspot assessment that were relevant for the unit processes included in the system boundaries. The final choice was based on the relevance of the company’s own code of conduct and supplier code of conduct, the requirements of the SAQ 4.0 (Sustainability 2021), customer requirements on human rights due diligence in the supply chain, and legal requirements, such as from the German Act on Corporate Due Diligence Obligations in Supply Chains. The latter, for example, addresses child labor, forced labor, slavery, occupational health and safety, freedom of associations, discrimination, fair salary, harmful soil change, pollution, unlawful seizure of land, and security forces (Bundestag 2021). The stakeholder category workers, value chain actors, society, and local community were chosen for the study. Twelve related subcategories were assessed by 22 social indicators (mainly taken from the methodological sheets), whereby the assignment of the social indicators to the respective subcategory varies between one and three social indicators.
Table 1 shows the stakeholder categories, subcategories, and social indicators with the units considered in this study. In addition, the data quality scores using the quality indicators from the pedigree matrix with 1 indicating the best and 5 indicating the worst data quality (covering reliability, completeness, time, geography, and technology) (Eisfeld 2017).Table 1 Overview of all indicators considered in the analysis with their selection criteria and the respective data quality based on the PSILCA© pedigree matrix assessment. The indicators marked with * were evaluated by the authors of this study according to the pedigree evaluation
4.5 Modelling and social hotspot evaluation process
The next step was to allocate the information about the product systems and their boundaries to the social indicators from the PSILCA© database on sector and country level.
Activity variables display the process activities that can be assigned to the process output. In this study, the activity variables used were sector-based worker hours provided in the PSILCA© database. In PSILCA©, the worker hours are linked to 1 USD of process output using the following formula given in Eisfeldt (2017):
𝑊𝑜𝑟𝑘𝑒𝑟ℎ𝑜𝑢𝑟𝑠=𝑈𝑛𝑖𝑡𝑙𝑎𝑏𝑜𝑟𝑐𝑜𝑠𝑡𝑠𝑀𝑒𝑎𝑛ℎ𝑜𝑢𝑟𝑙𝑦𝑙𝑎𝑏𝑜𝑟𝑐𝑜𝑠𝑡(𝑝𝑒𝑟𝑒𝑚𝑝𝑙𝑜𝑦𝑒𝑒)
with𝑈𝑛𝑖𝑡𝑙𝑎𝑏𝑜𝑟𝑐𝑜𝑠𝑡𝑠=𝐶𝑜𝑚𝑝𝑒𝑛𝑠𝑎𝑡𝑖𝑜𝑛𝑜𝑓𝑒𝑚𝑝𝑙𝑜𝑦𝑒𝑒𝑠(𝑖𝑛𝑈𝑆𝐷𝑝𝑒𝑟𝑐𝑜𝑢𝑛𝑡𝑟𝑦−𝑠𝑝𝑒𝑐𝑖𝑓𝑖𝑐𝑠𝑒𝑐𝑡𝑜𝑟𝑎𝑛𝑑𝑦𝑒𝑎𝑟)𝐺𝑟𝑜𝑠𝑠𝑜𝑢𝑡𝑝𝑢𝑡(𝑖𝑛𝑈𝑆𝐷𝑝𝑒𝑟𝑐𝑜𝑢𝑛𝑡𝑟𝑦−𝑠𝑒𝑐𝑡𝑜𝑟𝑎𝑛𝑑𝑦𝑒𝑎𝑟)
In simpler terms, the number of worker hours reflects how many labor hours per USD are required to complete a production step in a specific sector (UNEP 2020).
Medium risk hours are the equivalents for specific social risks in the PSILCA© database. The more worker hours are worked in a sector and the higher the likelihood for local social risk, the higher the number of medium risk hours in a sector respectively the regarded supply chain. The medium risk hours are provided and calculated by PSILCA©, which specify the observed indicator risk related to its medium risk to produce 1 USD output of the assessed sector. Additionally, when considering value chains, the medium risk hours can also be offset against the monetary outputs of the respective value creation levels. This offsetting makes it possible to calculate a weighted distribution of social risks based on the monetary output on a sectoral level (Eisfeld 2017; Mancini et al. 2018).
All information available in PSILCA© V2 (Eisfeldt 2017) about the selected stakeholders, their impact categories, and the associated social indicators were assigned to the individual supply chain actor in OpenLCA by selecting a sector-specific process in the respective country. The most equivalent sectors were selected for each process and its specific country. If there was no directly matching sector for a process in PSILCA©, the next most similar sector was selected (e.g., for bauxite mining in Brazil, the iron mining sector in Brazil was selected). When interpreting the results, it was essential to note that not all the social indicators provided were related to the respective sector and were partially extrapolated or only provided on the country level. This circumstance was considered by evaluating the social indicators’ data quality.
In the results, two different evaluation methods were used for the stakeholder categories and the associated indicators. On the one hand, the medium risk hours described were used for “workers,” as these represent the potential social risks for workers most accurately. On the other hand, medium risk hours were not used for “local community,” “value chain actors,” and “society.” The indicators for the above stakeholder categories were primarily based on country level. An assignment of these indicators to sector-specific worker hours would thus lead to a misrepresentation of the risk potential. Hence, the approach of direct quantification of indicators in PSILCA©'s life cycle calculation developed by Maister et al. (2020) was used here.
Since PSILCA© offsets the worker hours per USD output specific to each sector and country with the assigned social indicators, it was necessary to assign a monetary input and output to each sector-specific value created in PSILCA© to calculate the medium risk hours for “workers”. Two approaches were adopted in the step of weighing the monetary output of each process with the respective social indicators. In the first approach, weighted values from the analysis of the output of each supply chain process were used. The weighting was done in each case with the determined market prices per 1 t of material. The monetary output was then allocated against the worker hours, giving a weighted distribution of social risks for the respective value chain in the form of medium risk hours. The other method used an unweighted approach. In this approach, each process was attributed to an output of one USD regardless of how much value was created at each value chain level. This method did not aggregate the medium risk hours output based on monetary values in PSILCA© and provided an unweighted comparison of the social indicators of the different countries and processes. The medium risk hours of the two weighting methods were presented in the results.
For the stakeholder category “Workers” results, a risk matrix was developed by the authors, which was used to show the correlation between sector-specific worker hours and the likelihood of the potential occurrence of the respective social indicator. This diagram represents a visualization of the unweighted medium risk hours calculation, i.e., the combination of the likelihood of a social risk with the respective worker hours for one USD of the sector. Figure 3 gives an overview of the steps of the social indicator analysis in this study.
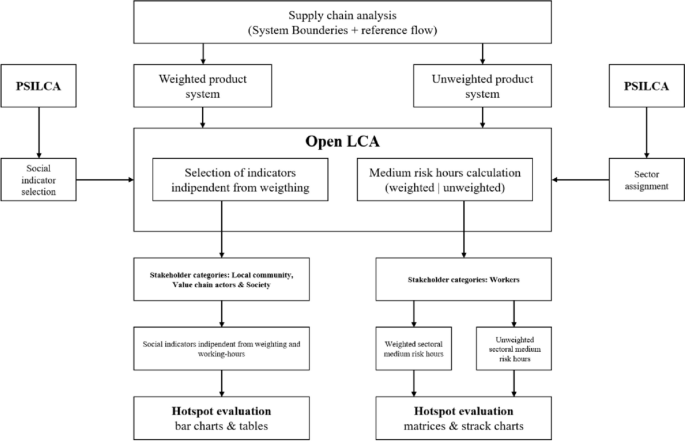
5 Results and discussion
5.1 Worker
Figure 4a shows the weighted medium risk hours (MHRs) based on the monetary output of the same processes—showing significant differences. The highest value was found for “AC1 Mg-Processing” (112.6 MRHs), directly followed by “EC2 Al-Tense scrap” (111.23 MRHs). For the weighted results, the contribution of the gender wage gap besides fair salary and child labor became a relevant contribution. The distribution of the MRHs for women in the sectoral labor force was about equal in all processes considered. Figure 4b indicates the unweighted MRHs of the eight supply chain actors from “Balancing disks.” The process “AC1 Mg-Processing” resulted in 5.36 MRHs when not weighted. The main contributors were fair salary and child labor. The second highest score was about 85% smaller and was found for “AC1 Si-Processing” (0.75 MRHs), where fair salary and child labor each approximately contributed half. The MRHs of the remaining processes ranged from 0.06 to 0.16.
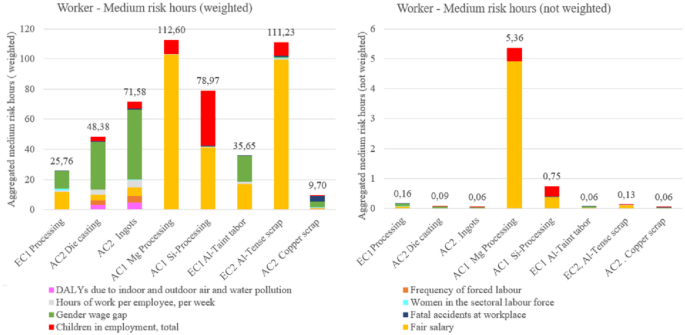
For “High purity aluminum,” the process “AfC1 Mining (Bauxite)” (Guinea) obtained the highest value (8.75 MRHs) when not weighted, mainly due to DALYs, fair salary, and child labor (Fig. 5b). The next lowest value, about 30% smaller, was found for “EC5 Mining (Bauxite)” (6.20 MRHs), almost equally composed of women in the sectoral labor force and gender wage gap. Also “NA Refinery (Al-Oxide)” showed a higher risk (2.80 MRHs) than the remaining processes (0.67–0.10 MRHs). When weighted, it was notable that the process “EC4 Smelting (Electrolysis)” was weighted much higher than the other processes in this supply chain with (902.87 MRHs), mainly because of the gender wage gap. The distribution of the total value for the required raw materials bauxite and aluminum hydroxide to the supply chain actors of mining and refinery led to significantly lower MRHs in the weighted consideration.
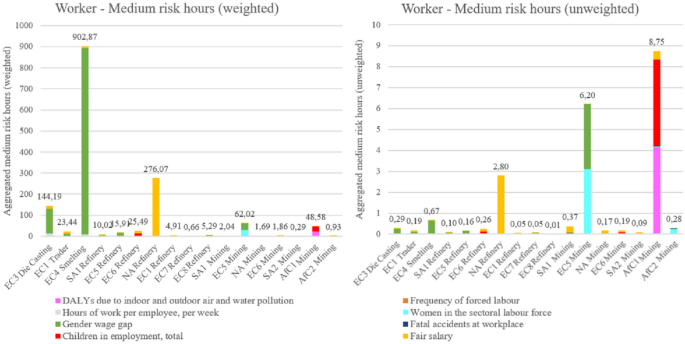
Matrices were developed by the authors to visualize potential social hotspots. Exemplarily, Fig. 6 shows the ratio of worker hours to the indicator children in employment, total. For “Balancing disks,” the processes from AC2 and AC1 are plotted in the red social hotspot area and the processes from AC2 are plotted in the medium risk range. For “High purity aluminum,” the processes from “AfC1 Mining (Bauxite)” and EC6 are plotted in the red social hotspot area. “SA2 Mining (Bauxite)” (Guyana) had a children in the employment rate of over 10% but a lower value for the worker hours and was plotted in the special focus area. “NA Mining (Bauxite)” and “NA Refinery (Al-Oxide),” “AfC2 Mining (Bauxite)” (Suriname), “SA1 Mining (Bauxite)” (Brazil) and internal processing in EC3 were in the medium risk area. However, the medium risk located processes represented only a small proportion of the MRHs. The MRHs in this supply chain for the indicator children in employment were dominated by bauxite mining in Guinea, representing the highest value with 38% of working children between 7 and 14 years. Here, it needs to be noticed that processes with very high MRHs shares could suppress the potential social risk identification of countries. The risk matrix helps to indicate that the shares for child labor of the processes in EC6 and Guyana were also indicated as a social risk.
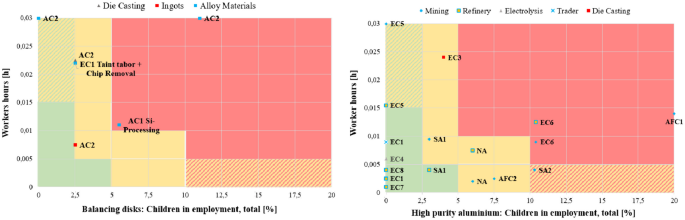
Across both supply chains, the processes in AC1, EC2, EC6, Guinea (AfC1), and Guyana (SA2) were in the area of a social hotspot for child labor. According to (Vasters and Franken 2020), artisanal mining methods rarely exist in the bauxite mining sector, and bauxite mining is only profitable through large-scale industrial mining using heavy equipment (Bergsdal et al. 2004). A low occurrence of child labor was expected for the industrial mining processes in EC6 and Guinea (AfC1). The assumption was also confirmed by the recent report of the non-governmental organization Human Rights Watch on bauxite mining which did not allege any child labor in this area (Wormington and Bugalski 2021).
No social hotspot was identified for frequency of forced labor for “Balancing disks” while the AC2 processes fell into the medium risk range. This distribution, however, was not detected in the unweighted MRHs as the values of the two AC1 processes showed an increased number of MRHs due to their higher number of worker hours. For the weighted assessment, due to the higher monetary output, this distribution changed (Fig. 7). For “High purity aluminum,” no social hotspot was found for frequency forced labor. The mining process in Guinea (AfC1) and the mining and refinery process from EC6 showed a medium risk.
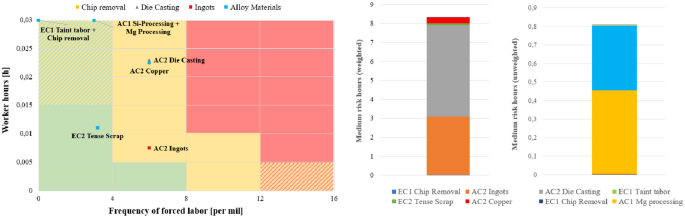
No social risk in both supply chains was identified for hours of work per employee, per week for both supply chains. Except for the working hours in the process in EC2 and the EC1 processes for “Balancing disk” that indicated low risk, all other processes were in the medium risk range. For “High purity aluminum,” only Guyana was on the borderline of the medium risk range. A similar distribution was detected for the unweighted MRHs for both supply chains.
For women in the sectoral labor force in the supply chain “Balancing disks,” the two processes located in EC1 revealed a social hotspot due to the general risk of unequal occupation for men and women in the respective country. Except for the copper recycling process in AC2 (medium risk), all other processes showed no risk. When unweighted, the processes from EC1 and the AC2 copper recycling made up the highest proportion. The distribution of the without social risk was roughly similar. For “High purity aluminum,” social hotspots were found for the mining sector in Guinea and EC5 while the mining sector in EC5 dominated the distribution of the unweighted MRHs.
For the gender wage gap, no information was available for several countries. The processes in AC2 and EC1 for “Balancing disks,” however, revealed social hotspots (except for the die casting process (AC2, medium risk)). The processes in EC1 had the highest average percentage pay gap for women compared to men. The high value for the company’s internal machining process was also reflected in the unweighted MRHs while the copper recycling process in AC2 had the lowest MRHs. For “High purity aluminum,” all processes indicated a social hotspot. The distribution of the available values suggested a tendency for a gender wage gap of 20–30%. The high value of 48.53% in the EC5 mining sector should be considered an extreme value that requires a more detailed investigation as the average salary gap in EC5 was around 8% according to the EU Report 2014 (Boll and Lagemann 2018). In 2014, the average salary gap between women to men was 14.1% in the EU and 20.1% in EC1 (Boll and Lagemann 2018). It could be concluded that the identified pay gaps were not a typical phenomenon for the sectors in this supply chain but rather a global task for society to close the pay gap between women and men.
Social hotspot for fatal accidents at workplace of the supply chain “Balancing disks” was the process of copper recycling in AC2 with 15.01 fatal accidents at the workplace per year and 100 k employees. It formed the highest proportion of the weighted and unweighted MRHs. For “High purity aluminum,” the processes in European countries overall showed no risk. The Brazil and Guinea mining sectors had values in the medium risk area. The mining sector in Suriname was the outlier in this indicator with a value of 33 fatal accidents per hundred thousand workers per year. The constellation of the three processes in Guinea, Suriname, and Brazil also represented the most significant proportions within the unweighted MRHs. It should be noted that social hotspots in Suriname, Brazil (SA1), and AC2 should only be used with reservation as these indicators were already more than 5 years old when the database was created in 2017 (Eisfeldt 2017).
Fairy salary in the supply chain “Balancing disks” sowed a medium risk for the AC1 silicon processing and EC2 recycling sectors. Only “AC1 Mg-Processing” indicated a social hot spot area. The remaining processes from AC2 and EC1 were placed in the no risk area. This distribution could also be seen in the unweighted MRHs. For “High purity aluminum,” “NA Refinery (Al-Oxide)” revealed a social hotspot. “NA Refinery (Al-Oxide)” had the highest value of unweighted and weighted MRHs. Except for the mining process in NA (medium risk), all processes were in the no risk range. For fair salary, PSILCA© used the calculation of the ratio between the two indicators, sector average wage, per month and living wage, per month. When calculating the unweighted MRHs for “High purity aluminum,” the increased number of MRHs for the mining process in Guinea was noticeable which was not covered by the position of Guinea in the risk matrix. Thus, an error in the calculation of MRHs for the process in Guinea by PSILCA© was assumed. This again demonstrated the need to regard risk factors from at least two perspectives.
Workers’ rights were analyzed separately from the worker hours. For the AC1 processes in the “Balancing disks” supply chain, the values for the right of association and the right to strike indicated social hotspots. The same applied to the AC2 copper recycling process, where the right of collective bargaining and the right to strike were in the red social hotspot area. The other processes mainly revealed no social risk with medium risk exceptions for Brazil (SA1) and AC2 (Table 2). The subcategory did not represent a reliable source of information with an inferior data quality basis. Also Mancini et al. (2018) refrained from using the workers’ rights information from PSILCA© in their work because of the low data quality. However, the results of these indicators were included for completeness.Table 2 Social hotspots for “workers’ rights” for both supply chains. 1 indicates the highest risk (major restrictions), and 3 indicates the lowest risk (no restrictions)
5.2 Society
The external fragile state index (Messner and Fiertz 2019) was used to assess the conflict risks of the countries in which the manufacturing processes took place. It evaluated the processes using the risk classification of Mancini et al. (2020) as shown in Fig. 8. Only the countries EC1 and EC7 revealed no risks in both supply chains. EC4 and EC8 showed a medium risk while the remaining countries indicated social hotspots. The country with the highest index value was Guinea (AfC1) in the “High purity aluminum” supply chain (97.4).
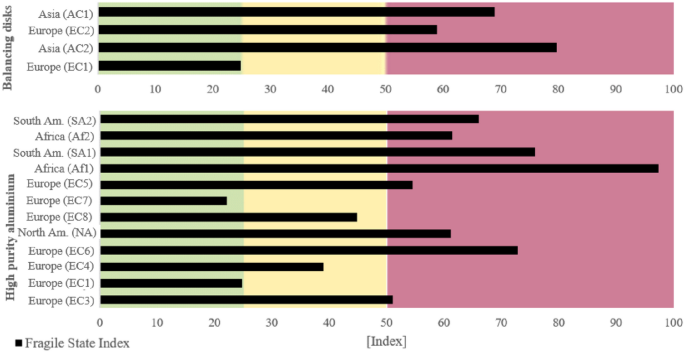
For internationally operating companies, it is essential to have an overview of the vulnerability of the countries and societies in their supply chains regarding the risk of state fragility to react quickly to changing political developments if necessary. In this study, 10 out of 16 countries indicated social hotspots for the fragile state index. In the case of the most fragile country of both supply chains in the index, a military-led coup against the current president occurred while conducting this work (Akinwotu 2021). Therefore, this incident was an excellent example of the potential impact of political instability on commodity markets (FT 2021). This index can help make purchasing and investment decisions. Nevertheless, the influence of an individual company in regions without any production facilities of their own or direct purchasing agreements was to be considered marginal.
5.3 Value chain actors
For presence of anti-competitive behavior or violation of anti-trust and monopoly legislation mining processes (Bauxite) as well as refinery processes (Al-Oxide) in EC1, EC6, and NA were identified as social hotspots. In the country-specific analysis of public sector corruption in only two countries in both supply chains—EC7 (73%) and EC1 (83%)—had values in the medium or no risk range (Fig. 9). All other countries indicated social hotspots. The worst-performing country was Guinea (AfC1, 27%). In the area of active involvement in corruption and bribery identified on sector level, the Brazil (SA1) and EC5 mining sectors performed the worst, each with a 19% share. Except for the EC1 trade sector (4%), the other sectors in both supply chains reached values of 8%.
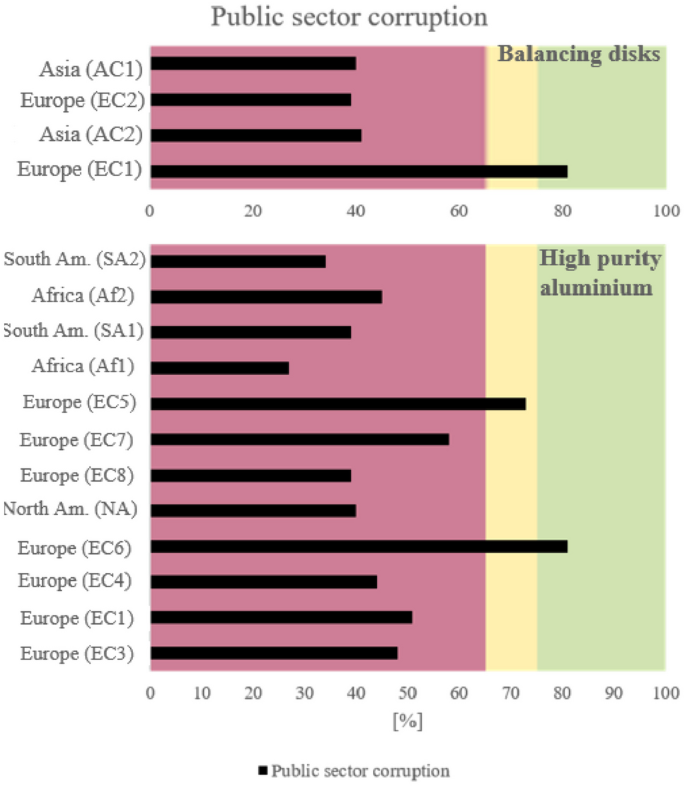
Regarding Guinea (AfC1), the most negative outlier in both supply chains, the statement on high public sector corruption was confirmed by literature sources, especially regarding bauxite mining in Guinea (Knierzinger 2014; Wilhelm and Maconachie 2021). The negative example of Guinea showed that the country-based indicator on public sector corruption in combination with literature research could provide detailed insights into processes taking place in countries if data sources were available. The subject area of the indicator presence of anti-competitive behavior was only covered for all values by extrapolation from U.S. sectors to global process-specific sectors. Overall, mining processes perform poorly. Since extrapolation was made from the US mining sector to the worldwide mining sectors, all figures were approximately the same. Due to the similar values, it was difficult to derive risks in the supply chain for this indicator.
5.4 Local community
In terms of respect of indigenous rights, no country indicated social hotspots as shown in Table 3. Contrary to our results, Rorato et al. (2020) demonstrated that mining in Brazil (SA1) is driving massive deforestation in virgin forest areas and is supported by the Brazilian government. It is significantly increasing the vulnerability of indigenous populations through expanded exploration activities. The report on the threat posed to local people in Guinea (AfC1) by certain bauxite mines also showed the increased risk that bauxite mining could pose (Wormington and Bugalski 2021). These examples strengthened that mere reliance on indicators provided by databases should never be used as the single source of decision-making. Verification by external independent literature sources or reports was crucial in this case.Table 3 Results of the presence of indigenous peoples within the supply chain and how the respective countries deal with their presence. N.B. 3 indicates medium risk and 5 indicates no risk.
In terms of environmental aspects and the related impact categories pollution level, drinking water coverage, and sanitation coverage, errors were found in the raw data provided by PSILCA. Hence, the external Environmental performance index (EPI) indicator was chosen as the pollution level substitute (Wendling et al. 2020). The drinking water and sanitation coverage indicators also listed in the EPI were used. In the overall EPI, AC1 (37.3%), EC2 (49%), AC2 (42.6%), Guyana (SA2, 35.9%), Suriname (AfC2, 45.2%), NA (48.2%), and EC6 (45.4%) were placed in the hotspot range. Guinea's environmental performance (AfC1, 26%) was significantly lower than that of the other countries in the hotspot area. EC3 (63.7%), EC4 (68.2%), EC8 (74.8%), EC7 (72.8%), and EC5 (69.1) were all in the medium risk range. EC1 performed best in the overall performance with 77.2%.
The EPI total score combined with the results from the sanitation coverage and drinking water coverage indicators revealed a tendency to derive potential environmental hazards due to company activities, as literature sources and reports on Brazil and Guinea countries confirmed (Keita 2020; Fouche 2021; Wormington and Bugalski 2021). Moreover, it should be noted that the red mud generated during alumina production poses a tremendous environmental risk if not properly processed (Sushil and Batra 2008).
5.5 Overall findings
For “Balancing disks,” a total of 7 social hotspots were identified for the workers and 10 for “High purity aluminum.” For the fragile state index, 3 of 4 actors were identified in the social hotspot area in the secondary route and 7 of 11 actors were identified in the primary one. For the EPI, the secondary route also indicated 3 out of 4 actors in the social hotspot area while the primary route came up with 5 out of 11 actors. For the indicators of the value chain actors, similar distributions as for the fragile state index emerged. In both supply chains, processes in AC1 and Guinea (AfC1) were most frequently in the social hotspot range across all stakeholder categories. While more social hotspots for “High purity aluminum” route were found, the percentage of social hotspots in the’Balancing disks’ route was higher across all processes. This is because of the shorter supply chain of the “Balancing disks” (beginning on the Tier 4 supplier level). For this supply chain, seven supply chain actors in three different countries were considered before the company’s internal production while there were 24 actors in eleven countries in the “High purity aluminum” supply chain. The difference in the number of supply chain actors and countries alone implied a higher complexity for further investigations primary route. The potential for social hotspots may be increased due to the higher number of different countries. A statement that secondary material supply chains perform better in the social area than primary aluminum routes, as in the ecological footprint, could not be derived from the results presented.
5.6 Limitations
After creating the product system and the definition of the system boundary, a suitable country-specific sector was assigned to each of the identified process steps, representing the respective process in this country best. In making this selection, it was difficult to rely on existing scientific literature since an analysis of the aluminum sector using PSILCA has not yet taken place. The selected sectors could also be seen in each case as an approximation of the respective process taking place in the country. Overarching sectors, such as the chemical sector for the process of refining bauxite, were often selected. Aluminum-specific sector information was not available in any country. The data quality information provided by PSILCA through the pedigree matrix assessment for each social indicator helped to assess each indicator's usability. Indicators were not excluded from the analysis in advance if their data quality was considered very low compared to the other indicators to avoid misappropriation of results. In several country-specific sectors, gaps in data occurred in the results, which were not replaced by sector information from adjacent countries. A country substitution was not applied to avoid a possible unjustified social hotspot classification of a country or supplier.
In some cases, in the raw data, values were given for “Drinking water coverage” and “Sanitation coverage” in certain countries, which were commented as “not available” by PSILCA©. Due to this uncertainty in usability, the environmental performance index, an external environmental indicator, was used, and the data from PSILCA© were excluded. Also for the risk of conflicts indicator, for which no output figures were provided by PSILCA©, an independent indicator was used with the fragile state index.
For “Workers,” the activity variable worker hours was applied to calculate the average medium risk hours. Two weighting methods were applied, one method to look at the weighted monetary output of the MRHs and the other to look at the unweighted MRHs without monetary weighting for better comparability of the different processes. The method of presentation with the weighted monetary output reflected the actual outputs generated at each supplier level. The biggest problem with the monetary weighting option was the distortion of potential social risks based on the MRHs alone. It transferred countries or sectors into potential hotspot categories they did not belong to based on their raw data. Looking at the unweighted MRHs, much less deflection of risk hours could be seen. This distribution also showed the lower weighting of the supply chain actors in the refineries and the bauxite mines. Hence, a lower weighting on these supplier levels could lead to bias in assessing social hotspots.
Last, the limited transparency due to the anonymization of country names for confidentiality issues needs to be stated. While the company can internally use the findings of the study, other researchers might not be able to verify or use all the information presented. With the German Act on Corporate Due Diligence Obligations in Supply Chains and related reporting requirements, more transparency also for external people to companies can be expected.
6 Conclusion and outlook
Karlewski et al. (2019) already noted that S-LCA is feasible in industries with complex supply chains such as the automotive industry. This study moreover confirmed the applicability of social hotspot assessments based on the UNEP 2020 Guidelines to conduct a risk assessment for the main focus areas of the German Act on Corporate Due Diligence Obligations in Supply Chains such as child labor. However, the implementation of the work was associated with an enormous effort in the area of information collection about the supply chains to be investigated. This process depended on the direct suppliers’ willingness and was only feasible with their help. The guidelines used in combination with the associated methodological sheets were beneficial in structuring the procedure within the social hotspot analysis—giving the inexperienced user a guide. The social hotspot analysis was conducted with the help of the social indicator database PSILCA©. This facilitated data collection and saved much time compared to a fully primary data collection. Combining primary company data with generic data from the PSILCA© database thus proved to be a good combination in this regard. Still, PSILCA© should be used with attention due to data inconsistencies recognized in this work.
The results showed that in the areas of both investigated aluminum supply chains in the upstream area, the highest number of social hotspots occurred outside Europe across the different investigated indicators. The most hotspots were identified in Guinea, EC6, and AC1 in the stakeholder categories “Workers” and “Local community.” Significantly, the processes of alloy element production in AC1 and the bauxite mining in Guinea had an above-average number of social hotspots compared to the other supply chain actors.
The findings of social hotspot analyses can be used by the aluminum company to help their identified partners to enhance their social performance, for example by providing training and more opportunities through close collaborations or providing safety technologies. A shift of production locations, however, might technically not be feasible or even lead to other negative social impacts (e.g., job losses). The dialogues with suppliers have shown that cooperation on sustainability in complex supply chains is possible if it takes place on an equal level in an environment of trust. The company will use the findings and recommendations resulting from this work to improve its due diligence system along its supply chains that allows identifying supply chain actors in a secure data environment to generate sustainability along the complex automotive supply chains. The industry, particularly the automotive industry, should pursue the standardization of indicators and systems for sustainability evaluation to create a comparative framework that is justifiable and applicable to all stakeholders in the long term.
In the past, social assessments have only calculated the likelihood of a particular social risk without the link to activity variables. Using worker hours as an activity variable to measure the impact within supply chains created another dimension for classifying social risks. In the area of the application of the activity variable, a need for scientific research arose concerning the provision of correction factors which, for example, can include the degree of automation of production sites in the activity variable to achieve more detailed information on the hours worked at the site. Standardization for the calculation of activity variables should also be further developed. Moreover, the formulation of accepted data quality criteria and data exclusion criteria can contribute to the comparability of social assessments. Scientific research and industry can contribute to further developing these recommendations to improve the level of detail of generic social assessments continuously.
Data availability
The data that support the findings of this study are available in the generic PSILCA© database.
References
- Akinwotu E (2021) Guinean soldiers claim to have seized power in coup attempt. In: Guard. https://www.theguardian.com/world/2021/sep/05/guinean-soldiers-claim-to-have-seized-power-in-coup-attempt
- Bergsdal H, Strømman AH, Hertwich EG (2004) The Aluminium Industry - Environment, Technology and Production. Norwegian University of Science and Technology (NTNU), Trondheim
- Boll C, Lagemann A (2018) Gender pay gap in EU countries based on SES (2014)
- Bruschi GJ, Fante F, de Araújo MT, et al (2021) Analysis of different failure criteria to evaluate bauxite tailingmechanical behavior through numerical modelling. Soils and Rocks 44:1–10. https://doi.org/10.28927/SR.2021.053720
- Bundestag (2021) bundestag.de. https://www.bundestag.de/dokumente/textarchiv/2021/kw23-de-lieferkettengesetz-845608
- Burger J (2014) Indigenous peoples, extractive industries and human rights : in-depth analysis.
- Cui J, Roven HJ (2010) Recycling of automotive aluminum. Trans Nonferrous Met Soc China (English Ed 20:2057–2063. https://doi.org/10.1016/S1003-6326(09)60417-9
- Deutsches Institut DIN, für Normung e.V. (2006) Umweltmanagement – Ökobilanz – Grundsätze und Rahmenbedingungen (ISO 14040:2006); Deutsche und Englische Fassung EN ISO 14040:2006. ICS 13:020Google Scholar
- Di Giorgio P, Petti L, D’Eusanio M, Serreli M (2022) Social life cycle assessment of Aluminium profile produced by Ponzio S.r.l. In: UNEP (ed) Pilot projects on Guidelines for Social Life Cycle Assessment of Products and Organizations. pp 64–75
- Doerr J, Ardey N, Mendl G, Fröhlich G, Straßer R, Laudenbach T (2019) The new full electric drivetrain of the Audi e-tron. In: Liebl J, Der Antrieb von morgen 2019. Springer Vieweg, Wiesbaden. https://doi.org/10.1007/978-3-658-26056-9_2
- Eisfeld F (2017) PSILCA – a Product Social Impact Life Cycle Assessment database Version 2.1. (G. GmbH, Ed.)
- Fouche G (2021) Brazil group sues Norsk Hydro over alleged pollution (Reuters, Ed.). https://www.reuters.com/article/us-norsk-hydro-brazil-idUSKBN2A923Q
- FT (2021) The coup in Guinea that shook the aluminium market. In: Financ. Times. https://www.ftc.gov/enforcement/cases-proceedings
- Karlewski H, Lehmann A, Ruhland K, Finkbeiner M (2019) A practical approach for social life cycle assessment in the automotive industry. Resources 8:. https://doi.org/10.3390/resources8030146
- Keita M (2020) Environmental impact of open PIT mining: case of bauxite mining in Guinea –a review. Int J Appl Environ Sci 15:167–177Google Scholar
- Knierzinger J (2014) The socio-political implications of bauxite mining in Guinea: a commodity chain perspective. Extr Ind Soc 1:20–27. https://doi.org/10.1016/j.exis.2014.01.005Article Google Scholar
- Kvande H (2011) Production of primary aluminium. In: Lumley R (ed) Fundamentals of aluminium metallurgy. Woodhead Publishing Limited, Cambridge, pp 49–69Chapter Google Scholar
- Liu DY, Wu CS (2012) Stockpiling and comprehensive utilization of red mud research progress. Materials (basel) 5:1232–1246. https://doi.org/10.3390/ma5071232Article CAS Google Scholar
- Luthin A, Backes JG, Traverso M (2021) A framework to identify environmental-economic trade-offs by combining life cycle assessment and life cycle costing – a case study of aluminium production. J Clean Prod 321:128902. https://doi.org/10.1016/j.jclepro.2021.128902Article CAS Google Scholar
- Mahinroosta M, Allahverdi A (2018) Hazardous aluminum dross characterization and recycling strategies: a critical review. J Environ Manage 223:452–468. https://doi.org/10.1016/j.jenvman.2018.06.068Article CAS Google Scholar
- Maister K, Di Noi C, Ciroth A, Srocka M (2020) PSILCA - A Product Social Impact Life Cycle Assessment database Version 3. Berlin: Green Delta GmbH. Retrieved August 23, 2021, from https://psilca.net/wpcontent/uploads/2020/06/PSILCA_documentation_v3.pdf
- Mancini L, Eynard U, Eisfeldt F, et al (2018) Social assessment of raw materials supply chains: a life-cycle-based analysis
- Mancini L, Eslava NA, Traverso M, Mathieux F (2020) Responsible and sustainable sourcing of battery raw materials
- Martin M, Herlaar S (2021) Environmental and social performance of valorizing waste wool for sweater production. Sustain Prod Consum 25:425–438. https://doi.org/10.1016/j.spc.2020.11.023Article Google Scholar
- Martin SC, Larivière C (2014) Community health risk assessment of primary aluminum smelter emissions. J Occup Environ Med 56:33–39. https://doi.org/10.1097/JOM.0000000000000135Article CAS Google Scholar
- Menzie WD, Barry JJ, Bleiwas DI, et al (2010) The global flow of aluminum from 2006 through 2025 Open-File Report 2010–1256. Sci a Chang World 1–78
- Messner J, Fiertz C (2019) Fragile states index annual report 2019. In: Washingt. D.C. Fund Peace. https://fragilestatesindex.org/wp-content/uploads/2019/03/9511904-fragilestatesindex.pdf
- Milovanoff A, Posen ID, MacLean HL (2021) Quantifying environmental impacts of primary aluminum ingot production and consumption : a trade-linked multilevel life cycle assessment. J Ind Ecol 25:67–78. https://doi.org/10.1111/jiec.13051Article CAS Google Scholar
- Rorato AC, Camara G, Escada MIS, et al (2020) Brazilian amazon indigenous peoples threatened by mining bill. Environ Res Lett 15:. https://doi.org/10.1088/1748-9326/abb428
- Schiessl A, Müller R, Volk R, Schultmann F (2021) Site-specific environmental impact assessment as a basis for supplier selections – exemplary application to aluminum. J Clean Prod 290:
- Sushil S, Batra VS (2008) Catalytic applications of red mud, an aluminium industry waste: a review. Appl Catal B Environ 81:64–77. https://doi.org/10.1016/j.apcatb.2007.12.002Article CAS Google Scholar
- Sustainability D (2021) https://www.drivesustainability.org.
- Thies C, Kieckhäfer K, Spengler TS, Sodhi MS (2019) Assessment of social sustainability hotspots in the supply chain of lithium-ion batteries. Procedia CIRP 80:292–297. https://doi.org/10.1016/j.procir.2018.12.009Article Google Scholar
- UNEP (2013) The methodological sheets for sub-categories in social life cycle assessment ( S-LCA ). Benoît Norris, C., Traverso, M., Valdivia, S., Vickery-Niederman, G., Franze, J., Azuero, L., Ciroth, A., Mazijn, B., Aulisio, D.
- UNEP (2020) Guidelines for social life cycle assessment of products and organizations 2020. Benoît Norris; C.; Traverso; M.; Neugebauer; S.; Ekener; E.; Schaubroeck; T.; Russo Garrido; Berger; Valdivia; Lehmann; A.; Finkbeiner; Arcese; G. (eds.). United Nations Environment Programme (UNEP).
- UNEP (2021) Methodological Sheets for Subcategories in Social Life Cycle Assessment (SLCA) 2021. In: Traverso M, Valdivia S, Luthin A, Roche L, Arcese G, Neugebauer S, Petti L, D’Eusanio M, Tragnone BM, Mankaa R, Hanafi J, Benoît Norris C, Zamagni A (eds) United Nations Environment Programme (UNEP)
- Vasters J, Franken G (2020) Aluminium: Informationen zur Nachhaltigkeit. Bgr 1–17
- Wallace G (2011) Production of secondary aluminium. In: Lumley R (ed) Fundamentals of aluminium metallurgy. Woodhead Publishing Limited, Cambridge, pp 70–82Chapter Google Scholar
- Wendling Z, Emerson J, de Sherbinin A, Esty D (2020) Environmental performance index. In: New Haven, CT Yale Cent. Environ. Law Policy
- Werker J, Wulf C, Zapp P et al (2019) Social LCA for rare earth NdFeB permanent magnets. Sustain Prod Consum 19:257–269. https://doi.org/10.1016/j.spc.2019.07.006Article Google Scholar
- Wesdock JC, Arnold IMF (2014) Occupational and environmental health in the aluminum industry: key points for health practitioners. J Occup Environ Med 56:5–11. https://doi.org/10.1097/JOM.0000000000000071Article Google Scholar
- Wilhelm C, Maconachie R (2021) Exploring local content in Guinea’s bauxite sector: Obstacles, opportunities and future trajectories. Resour Policy 71:101935. https://doi.org/10.1016/j.resourpol.2020.101935Article Google Scholar
- Wormington J, Bugalski N (2021) Aluminum: the car industry’s blind spot. In: New York Hum. Rights Watch. https://www.hrw.org/report/2021/07/22/aluminum-car-industrys-blind-spot/why-car-companies-should-address-human-rights
- Zimring CA (2016) Upcycling in history: is the past a prologue to a zero-waste future? The case of aluminum. In: Mauch C (ed) RCC Perspectives. Rachel Carson Center, pp 45–52
Acknowledgements
We would like to thank the aluminum company and their suppliers for their support and data provision to make this study feasible.
Funding
Open Access funding enabled and organized by Projekt DEAL.
Author information
Authors and Affiliations
- Institute of Sustainability in Civil Engineering, RWTH Aachen University, Aachen, GermanyAnna Luthin, Jan Knacksterdt & Marzia Traverso
- The University of Melbourne, Parkville, AustraliaAnna Luthin
Corresponding author
Ethics declarations
Conflict of interest
The authors declare no competing interests.
Additional information
Communicated by Sara Russo Garrido.
Publisher's Note
Springer Nature remains neutral with regard to jurisdictional claims in published maps and institutional affiliations.
Rights and permissions
Open Access This article is licensed under a Creative Commons Attribution 4.0 International License, which permits use, sharing, adaptation, distribution and reproduction in any medium or format, as long as you give appropriate credit to the original author(s) and the source, provide a link to the Creative Commons licence, and indicate if changes were made. The images or other third party material in this article are included in the article's Creative Commons licence, unless indicated otherwise in a credit line to the material. If material is not included in the article's Creative Commons licence and your intended use is not permitted by statutory regulation or exceeds the permitted use, you will need to obtain permission directly from the copyright holder. To view a copy of this licence, visit http://creativecommons.org/licenses/by/4.0/.
About this article
Cite this article
Luthin, A., Knacksterdt, J. & Traverso, M. Social hotspots in the automotive industry’s aluminum value chains—combining primary and generic data. Int J Life Cycle Assess (2024). https://doi.org/10.1007/s11367-024-02305-x
- Received26 April 2023
- Accepted24 March 2024
- Published26 April 2024
- DOIhttps://doi.org/10.1007/s11367-024-02305-x
Share this article
Anyone you share the following link with will be able to read this content:Get shareable link
Provided by the Springer Nature SharedIt content-sharing initiative