This introduction paper is based on the paper "Data Models for Casting Processes – Performances, Validations and Challenges" published by "IOP Publishing".
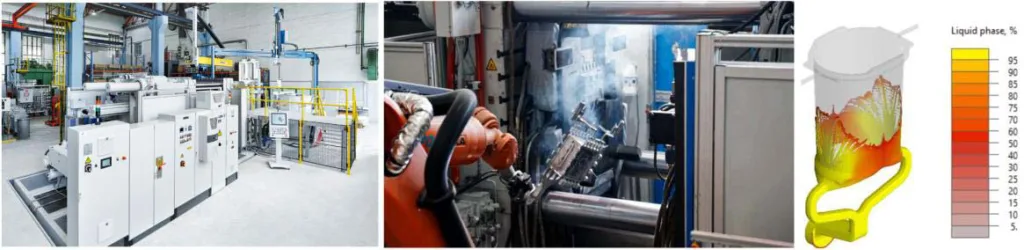
1. Overview:
- Title: Data Models for Casting Processes – Performances, Validations and Challenges
- Author: A M Horr, R Gómez Vázquez, and D Blacher
- Year of publication: 2024
- Journal/academic society of publication: IOP Conference Series: Materials Science and Engineering
- Keywords: Data-driven models, data learning, data training, light metal casting processes, vertical direct chill casting, HPDC applications, database building, data translations, sampling, real-time model building, validations, data solvers, interpolators, machine learning schemes.
2. Abstract:
Data-driven models with their associated data learning and training schemes can be utilised for the light metal casting processes. This paper presents the basis of data model building processes along with data training and learning exercises for vertical direct chill casting and high pressure die casting (HPDC) applications. The concepts of efficient database building, data translations and sampling, as well as real-time model building and validations are briefly discussed. Rigorous performance studies were additionally carried out for two real-world case studies. Different combinations of data solvers and interpolators are adapted for the model building techniques, while machine learning schemes are used for data trainings.
3. Introduction:
The data-driven and hybrid physical-data driven techniques are transforming the process modelling schemes toward more digitally oriented and cyber-based manufacturing processes. These digitalisation transformations promote more energy efficiency, productivity and innovation through faster and more effective modelling. The casting process is one of the most traditional material processes, which has been used to manufacture metallic and plastic parts. For the case of light metals like aluminium and magnesium, various casting processes are used to produce quality parts with reasonable strength [1]. The data real-time and reduced models can play a significant role in the digitalisation of these casting processes by increasing the pace of initial design and controlling of casting machines [2]. This would reduce the downtime preparations, and costly iterative trial and errors, while saving material and cost for new process designs [3]. There are various opportunities to improve the material processes like continuous direct chill casting, for instance, during planning of a new casting set up (e.g., alloy component) and/or choosing suitable set of parameters. Even though numerical simulations can help to optimise these processes, they are computationally expensive to evaluate the outcome for a single set of process parameters [4].
This paper presents an overview over the creation of efficient data models, their performances, validations, and challenges for casting processes. The role of appropriate combinations of data solvers and interpolators along with effects of data learning and trainings were examined and issues of applicability and reliability have been scrutinized. Two real-world case studies for vertical direct chill casting (VDCC) and multi-cycled high pressure die casting (HPDC) have been considered. The potentials for predictions and estimations of responses based on variations of process input parameters have been examined for further process optimisations and controlling.
4. Summary of the study:
Background of the research topic:
The study addresses the increasing importance of data-driven and hybrid physical-data driven techniques in transforming process modelling towards digitally oriented and cyber-based manufacturing. Casting, a traditional material process, is identified as an area where digitalisation through data models can enhance energy efficiency, productivity, and innovation.
Status of previous research:
While numerical simulations using sophisticated and multi-physical finite element (FE) and computational fluid dynamics (CFD) techniques are utilized for process modelling in casting, they are computationally expensive, especially for evaluating various process parameters. This limitation hinders rapid process optimisation and design.
Purpose of the study:
This paper aims to provide an overview of the creation, performance, validation, and challenges of efficient data models for casting processes. It investigates the role of data solvers and interpolators, the effects of data learning and training, and the applicability and reliability of data models in casting.
Core study:
The core of the study involves examining two real-world case studies: vertical direct chill casting (VDCC) and multi-cycled high pressure die casting (HPDC). The research explores the potential of data models to predict and estimate process responses by examining different combinations of data solvers and interpolators, and by employing machine learning schemes for data training. The study scrutinizes the performance, validation, and accuracy of these data models in the context of industrial casting applications.
5. Research Methodology
Research Design:
The research employs a case study approach, focusing on two distinct casting processes: vertical direct chill casting (VDCC) and high pressure die casting (HPDC). For VDCC, a fluid-thermal CFD simulation framework using the open-source solver directChillFoam was set up. For HPDC, simulations were performed using NovaFlow&Solid, a commercial CFD package. Data-driven modelling techniques were applied to both case studies.
Data Collection and Analysis Methods:
Off-line verified simulation results from CFD analyses were used to generate databases for data model building. For data analysis, various techniques were employed, including Singular Value Decomposition (SVD), Principal Component Analysis (PCA), regression, and clustering. Data interpolation methods such as Kriging, radial base function (RBF), and inverse distance (InvD) were utilized. Neural network schemes were also explored for data training. Performance was evaluated by comparing data model predictions with CFD simulation results, focusing on accuracy and reliability.
Research Topics and Scope:
The research focuses on building and validating data models for predicting temperature evolution in VDCC and HPDC processes. The scope includes:
- Database building for casting processes using CFD simulation data.
- Application of data solvers (SVD, PCA) and interpolators (Kriging, RBF, InvD) for model construction.
- Examination of data training using machine learning (neural networks).
- Performance evaluation and validation of data models against CFD simulations.
- Analysis of challenges and limitations in applying data models to casting processes, particularly concerning data sensitivity, database size, and validation rigor.
6. Key Results:
Key Results:
- Data models, particularly those using SVD combined with Kriging or InvD interpolators, demonstrate the potential for real-time temperature predictions in both VDCC and HPDC processes.
- For VDCC, data models showed reliable temperature predictions, but accuracy decreased in regions with high thermal gradients, such as near the solidification front.
- For HPDC, with a limited database size, data models could predict temperature trends but exhibited time shifts in cyclic temperature predictions, potentially due to challenges in predicting solidification time accurately.
- The study highlighted challenges in data model application to casting processes, including the need to handle rapidly changing data, the impact of database size and data sampling, and the rigor required for validation across different process conditions.
- Neural network training was suggested as a potential avenue for improving accuracy and reliability.
Figure Name List:
- Figure 1. schematic views of; a) vertical; b) horizontal and c) curved die chill casting processes
- Figure 2. HPDC machine at LKR laboratory with its typical numerical simulation
- Figure 3. Basic contour results used to generate main database; a) 2D contours from simulation scenarios; b) Data processing strategy to minimize sudden thermal discontinuities between rows; c) Side view of wedge domain.
- Figure 4. Typical CFD results for multi-cycle temperature time-history for HPDC process (six filling cycles)
- Figure 5. Temperature results for computational points along contour lines; a) DOE2 and; b) DOE3.
- Figure 6. Normalised error for computational points along contour lines; a) DOE2 and b) DOE3.
- Figure 7. Time-history temperature results for CFD and data models at; a) sensor 1 and; b) sensor 4
- Figure 8. Time-history temperature results with overlying descending temperature curves; a) sensor 1, DOE 1 and; b) sensor 4, DOE 2.
7. Conclusion:
The utilisation of data models for material process modelling can enhance the optimisation of existing processes and accelerate the design of future processes. In this paper, the performance, validation, and challenges related to these fast data models have been scrutinised to evaluate their accuracy and performances for material casting processes. In the first part of the manuscript, a brief representation of the direct chill casting and HPDC processes and their modelling and simulation techniques were illustrated, and some technical characteristics of these processes were discussed. The basic concept of casting processes' data models and their associated database building schemes were presented next, and the efficient use of data solver-interpolator combinations were discussed. In the following parts of the paper, two industrial case studies for VDCC and HPDC multi-cycle filling processes have been explained and their technical modelling challenges were examined. The performance, validations, and accuracy of process data models were scrutinised at the final part of manuscript, where major challenges related to the utilisation of these fast models were discussed.
From the presented results, it can be observed that proper combination of data solvers and interpolators can produce satisfactory results. However, the issues of high heating\cooling rates during casting processes can to some degree be problematic for the data models. Nevertheless, with further data training and learning, it is possible to achieve better results even for the processes with high gradient heating\cooling rates. The results of more detailed investigations on the best performance "solver-interpolator" combinations will be topic of a further publication.
8. References:
- [1] Luo, A. A. (2021). Recent advances in light metals and manufacturing for automotive applications. CIM Journal, 12(3), 79-87. https://doi.org/10.1080/19236026.2021.1947088.
- [2] Horr A. M. (2021). Optimization of Manufacturing Processes Using ML-Assisted Hybrid Technique, Journal of Manufacturing Letter, https://doi.org/10.1016/j.mfglet.2021.10.001.
- [3] Horr A. M. (2020). Notes on New Physical & Hybrid Modelling Trends for Material Process Simulations, Journal of Physics: Conference Series, 1603(1), https://doi.org/10.1088/1742-6596/1603/1/012008.
- [4] Weckman D.C., Niessen P. (1982). A numerical simulation of the D.C. continuous casting process including nucleate boiling heat transfer. Metall Trans B 13, 593-602. https://doi.org/10.1007/BF02650017.
- [5] Horr A. M, Kronsteiner J. (2021). Dynamic material process simulations: Hybrid-evolving technique, Journal of Metals, 11(12), 1884; https://doi.org/10.3390/met11121884.
- [6] Horr, A.M.; Kronsteiner, J. (2020). On Numerical Simulation of Casting in New Foundries: Dynamic Process Simulations. Metals, 10, 886. https://doi.org/10.3390/met10070886.
- [7] Lebon B. (2023). directChillFoam: an OpenFOAM application for direct-chill casting. Journal of Open Source Software, 8(82), 4871, https://doi.org/10.21105/joss.04871.
- [8] OpenFOAM-10 (2022). The OpenFOAM Foundation: https://openfoam.org.
- [9] Vreeman C.J., Schloz J.D. & Krane M.J.M. (2002). Direct Chill Casting of Aluminium Alloys: Modelling and Experiments on Industrial Scale Ingots, J. Heat Transfer 124 947-953. https://doi.org/10.1115/1.1482089.
- [10] directChillFoam Documentation. (2023). https://blebon.com/directChillFoam.
- [11] NovaFlow&Solid (2022). NovaCast Systems AB. https://www.novacast.se/product/novaflowsolid/.
9. Copyright:
- This material is a paper by "A M Horr et al". Based on "Data Models for Casting Processes – Performances, Validations and Challenges".
- Source of the paper: https://doi.org/10.1088/1757-899X/1315/1/012001
This material is summarized based on the above paper, and unauthorized use for commercial purposes is prohibited.
Copyright © 2025 CASTMAN. All rights reserved.