This introductory paper is the research content of the paper ["Correlation between process parameters and quality characteristics in aluminum high pressure die casting"] published by [NADCA].
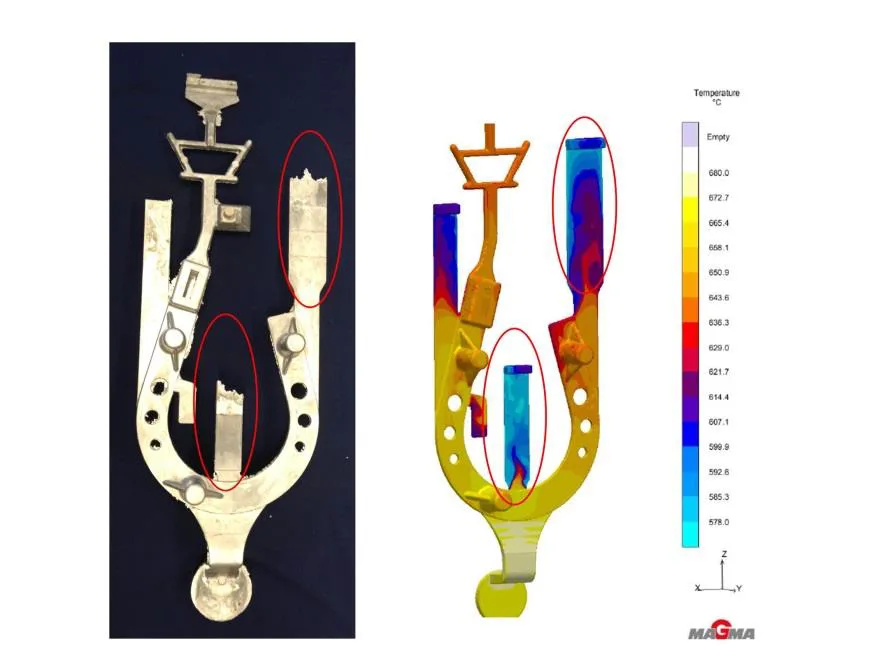
1. Overview:
- Title: Correlation between process parameters and quality characteristics in aluminum high pressure die casting
- Author: M. Winkler, L. Kallien, T. Feyertag
- Publication Year: 2015
- Published Journal/Society: DIE CASTING CONGRESS & EXPOSITION, NORTH AMERICAN DIE CASTING ASSOCIATION
- Keywords: (The paper does not explicitly list keywords, so this section cannot be populated based on the provided document.)
2. Abstract
Aluminum high pressure die casting is one of the most productive manufacturing processes. The complexity of the parts rises and the quality requirements are increasing. The challenge in high pressure die casting is to reach the high quality standards in spite of the huge number of quality influencing process parameters. The interaction of all quality influencing parameters leads to extremely high scrap rates of up to 10 - 25%. The parameters are not centrally monitored by one single unit but by the various systems of the process such as the die casting machine, the furnace, the thermal regulation system etc. The typical parameters being measured in the process to date are piston speed in the first and second phase, intensification pressure and others, but there are many parameters such as the humidity of the evacuated air which also control part quality.
The European research project MUSIC (MUlti-layers control and cognitive System to drive metal and plastic production line for Injected Components) has the aim to decrease the scrap rates in high pressure die casting by developing an intelligent cognitive system taking all quality controlling parameters into account. In the frame of the project a special casting geometry has been developed, that allows the production of parts with several defects such as shrinkage porosity, cold shuts and distortion. The die is instrumented with many new and innovative sensors to monitor new process parameters, such as the sound of the shot, which have not been applied to date. The sensor data, the process parameters of the machine and the peripheral devices are stored together with the quality index of the castings in one common database. The cognitive network will then be able to calculate the quality index for future parts based upon the measured sensor data.
3. Research Background:
Background of the research topic:
The number of high pressure die casting parts in the construction of the body of innovative cars is rising. The reason for the application of aluminum parts as structural elements is the reduction of the weight.
Status of previous research:
High pressure die casting (HPDC) is a productive manufacturing method, producing parts with good surface quality, high dimensional accuracy, and high tensile strength. However, a large number of parameters influence part quality. Conventional data acquisition (Figure 2) includes parameters like piston speed, switching point, intensification pressure, heating oil temperature, furnace temperature, and spraying unit parameters. These are typically controlled and measured by individual units. Other influencing parameters (e.g., humidity, evacuated air quantity, plunger acceleration, alloy composition variations) are not typically measured or stored.
Need for research:
The interactions between all quality influencing parameters are leading to high scrap rates up to 10 – 25%. This value exceeds the scrap rates of other manufacturing processes by a factor of 10 or even 1000.
4. Research purpose and research question:
Research purpose:
The European research project MUSIC (MUlti-layers control and cognitive System to drive metal and plastic production line for Injected Components) has the aim to decrease the scrap rates in high pressure die casting.
Core research:
To decrease the scrap rate by developing an intelligent cognitive system taking all quality controlling parameters into account.
5. Research methodology
The research utilizes a sensor network and cognitive system (Figure 3). A high pressure die casting machine and peripheral devices are equipped with sensors. All devices are attached to a network that is connected to one common data base. In this data base all sensor data and the controlled process parameters of the machine and the peripheral devices are stored.
A special casting geometry (Figure 4) was designed to intentionally produce defects (shrinkage porosity, cold shuts, distortion). The die is instrumented with sensors to monitor various process parameters.
To validate the final design of the cast part a filling and a solidification simulation was done with MAGMA.
The training of the cognitive model is the initial step for the prediction of the casting defects. Basis for this is the availability of process and quality data. The data are acquired during a design of experiments (DoE).
To analyze the same criteria of all castings a quality control procedure was defined.
6. Key research results:
Key research results and presented data analysis:
- A comparison of different cavity pressure signals is shown in Figure 8.
- The air mass sensor signal, combined with a vacuum system, is presented. Figure 10 shows a regular shot, while Figure 11 shows a shot with a leakage in the vacuum channel.
- Humidity sensor signals after long and short spraying cycles are shown in Figure 12.
- A correlation matrix (Table 1) was generated to show dependencies between parameters, sensor data, and quality results.
- An example of a 3D diagram from Design-Expert® software, illustrating interactions between quality index, temperature, and piston speed, is shown in Figure 13.
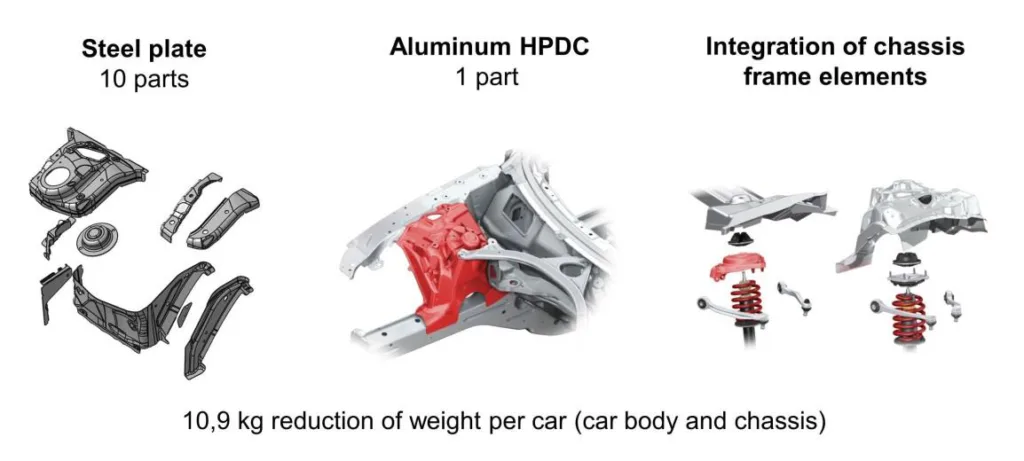
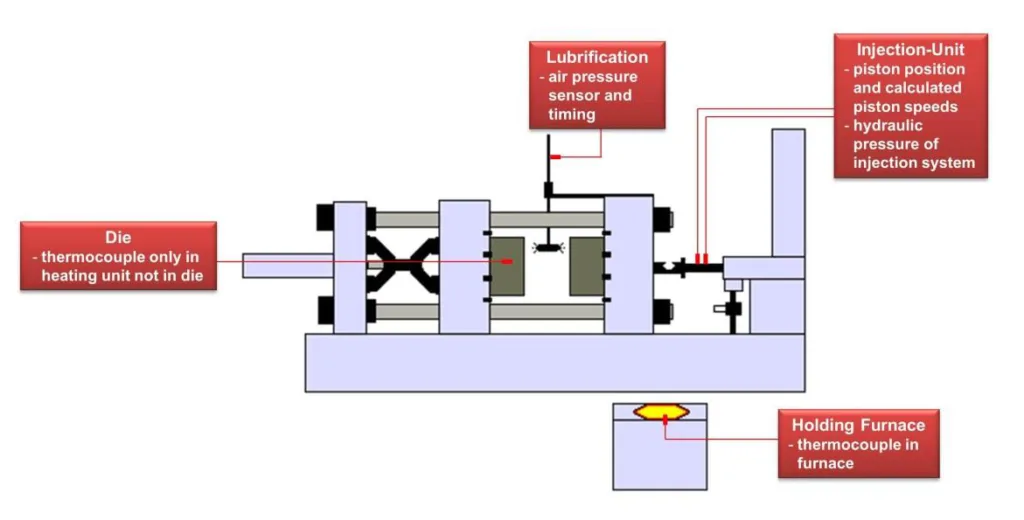
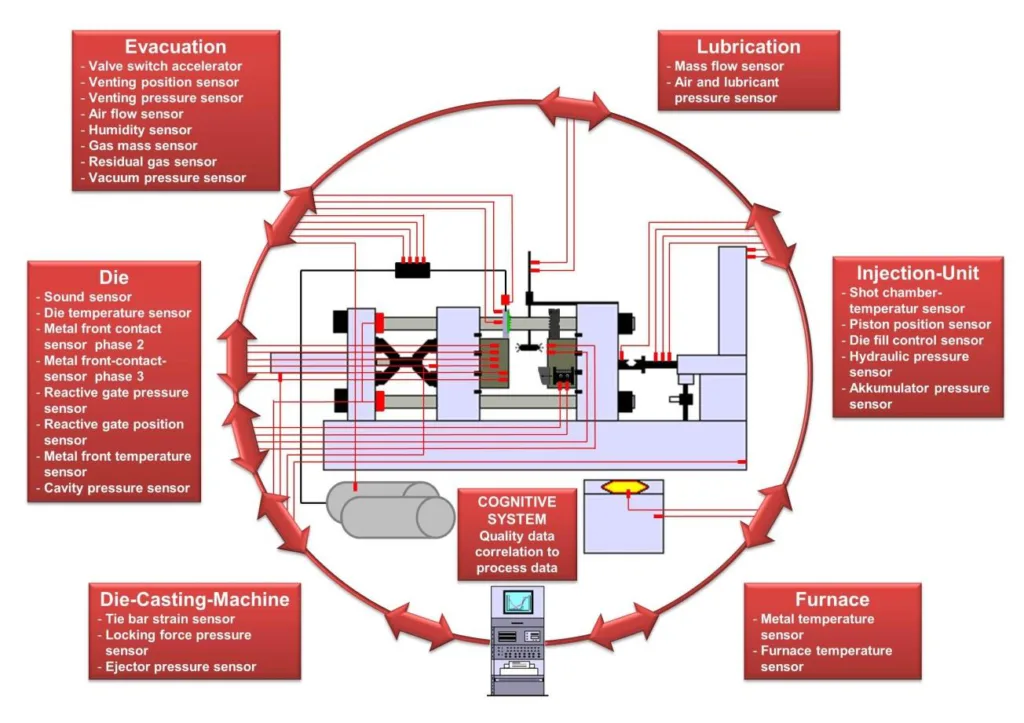
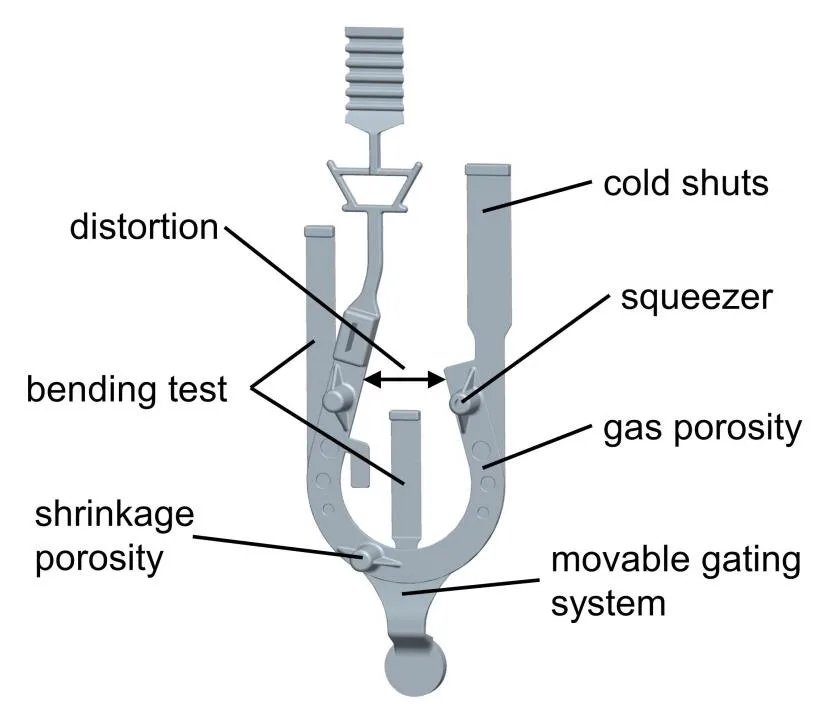
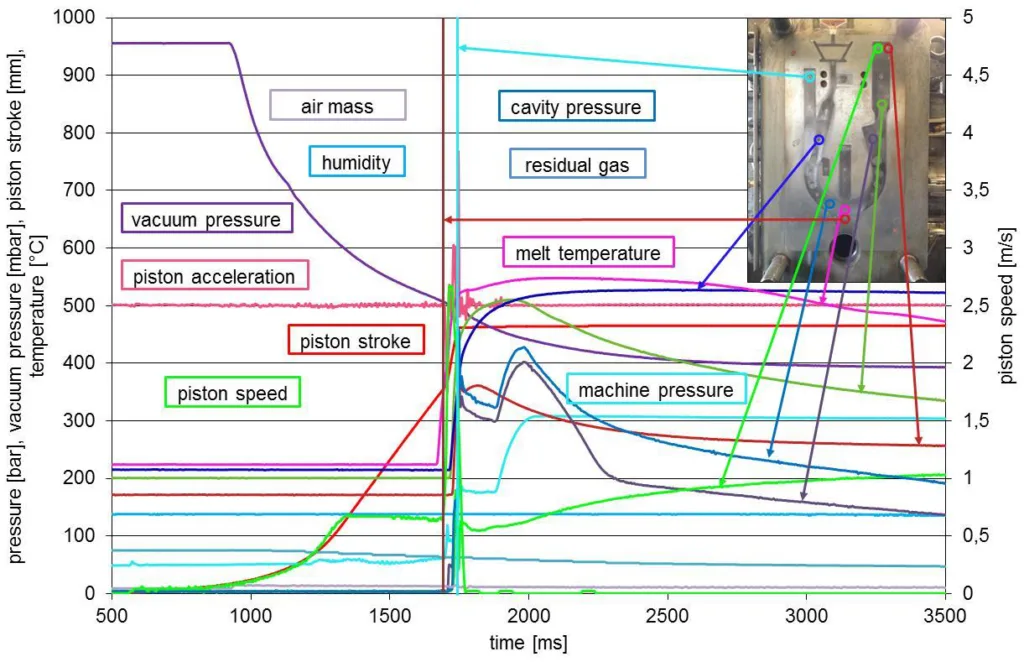
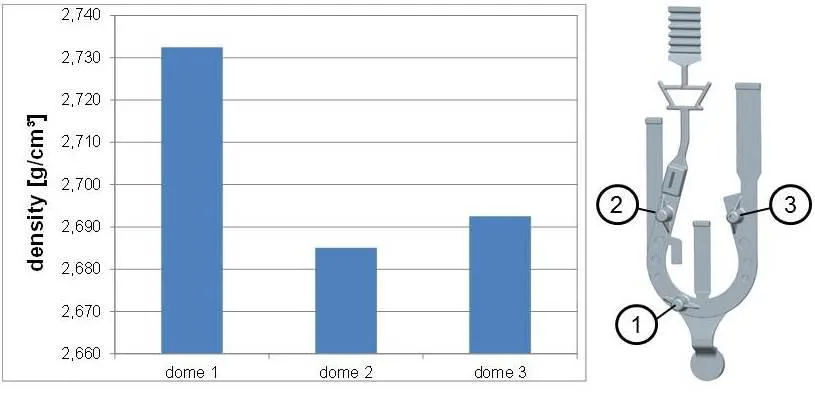
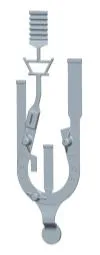
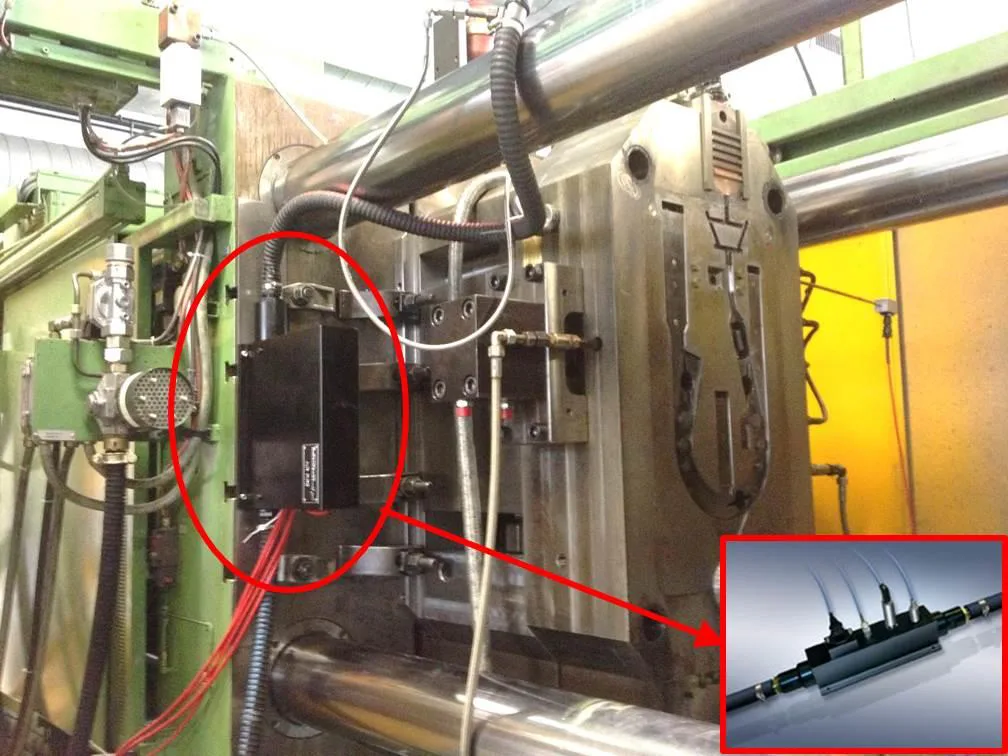
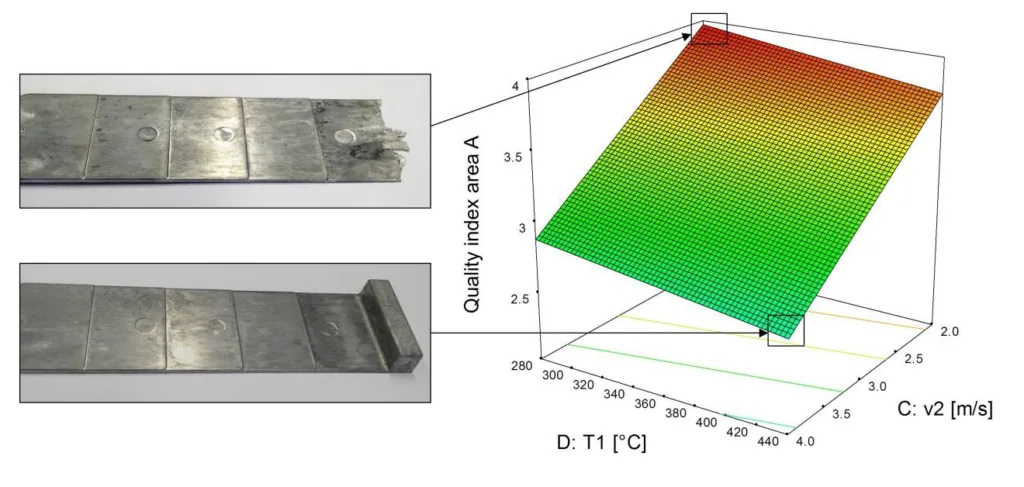
List of figure names:
- Figure 1: reduction of weight using structural aluminum high pressure die casting parts instead of joined steel parts [1]
- Figure 2: conventional data acquisition during HPDC process
- Figure 3: Innovative sensor network and cognitive system
- Figure 4: special casting geometry to produce defects
- Figure 5: Comparison of real cast part and simulation results regarding cold shuts [2]
- Figure 6: shot curve with additional sensor signals
- Figure 7: Comparison of the density of dome areas
- Figure 8: Shot curve with cavity pressure sensor signal with intensification pressure (orange color of sensor signals) and without intensification pressure (green color of sensor signals)
- Figure 9: Multi-airpipe-sensor-system assembled on the 750 t cold chamber machine of the foundry laboratory in Aalen [4]
- Figure 10: Shot curve with vacuum and air mass signal showing no leakage in the vacuum channel
- Figure 11: Shot curve with vacuum and air mass signal showing a leakage in the vacuum channel
- Figure 12: Shot curve with vacuum and humidity sensor signal showing measured after a long (blue) and a short (yellow) spraying cycle
- Figure 13: Interrelation between the quality index in area 'A', piston speed in the 2nd phase and temperature sensor signal T1
7. Conclusion:
Summary of key findings:
The cavity pressure sensors are monitoring the pressure in different regions of the casting. They provide information about the feeding efficiency and are helping to predict the density especially in thick areas of a cast part.
The air mass sensor allows measuring the amount of air that is evacuated and detects leakages in the vacuum channel. It helps to predict the density of a cast part with regard to entrapped air due to poor evacuation.
With the humidity sensor an improvement of the spraying cycle with a minimization of the remaining humidity in the die can be reached.
Correlations help to train the cognitive model and to predict the quality of the cast parts without quality investigation. This is a great opportunity to decrease the effort of quality controls and to reduce the scrap rates.
The connection of all devices via OPC-UA allows not only the acquisition of data but also to send input variables to the control of the HPDC machine and the peripheral devices. This means that the system will be able to regulate the process by adjusting certain quality influencing parameters within defined thresholds to optimize the process.
8. References:
- [1] Wanke, P., Innovative Strukturgusskonzepte bei AUDI, AUDI AG, Gießereikolloquium Hochschule Aalen, (May 9, 2012)
- [2] Kallien, L., Winkler, M., Kognitives System zur Reduzierung der Ausschussrate im Druckguss, Horizonte, (April/May 2015)
- [3] Bonollo, F., Gramegna, N., et al., The MUSIC guide to key-parameters in High Pressure Die Casting, MUSIC Consortium (2014)
- [4] http://www.electronics-gmbh.de/electronics/english/produkt_multisensor.htm
9. Copyright:
- This material is a paper by "[M. Winkler, L. Kallien, T. Feyertag]": Based on "[Correlation between process parameters and quality characteristics in aluminum high pressure die casting]".
- Source of paper: [The original document does not contain a DOI URL. ]
This material was created to introduce the above paper, and unauthorized use for commercial purposes is prohibited. Copyright © 2025 CASTMAN. All rights reserved.