This article introduces the paper"A runner-optimization design study of a die-casting die presented at the Journal of Materials Processing Technology".
1. Overview:
- Title: A runner-optimization design study of a die-casting die
- Author: C.C Tai, J.C Lin
- Publication Year: 1998
- Publishing Journal/Academic Society: Journal of Materials Processing Technology, Elsevier Science S.A.
- Keywords: Runner-optimization; Die-casting; Abductive network
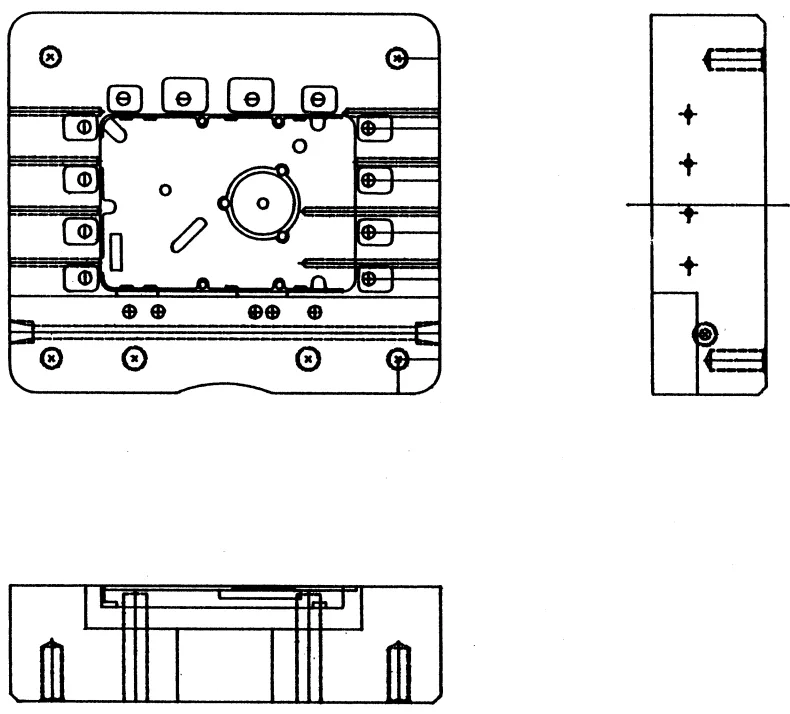
2. Research Background:
- Social/Academic Context of the Research Topic: Die casting is a widely used precise casting method in various industries such as automobiles, aerospace, and electronics due to its high strength and good appearance. The quality of die castings is critically determined by the gating system, particularly the runner design. Traditional die casting runner design involves repeated corrections during die-casting tests, leading to increased processing time and costs.
- Limitations of Existing Research: Previous research has focused on various aspects of die casting, including metal flow, cooling systems, solidification processes, and die life. However, there is a need for effective methods to optimize runner design to minimize residual stress and temperature, which are key factors affecting die life and casting quality. Existing methods for obtaining optimal die-casting parameters are often contradictory, making it challenging to maintain satisfactory casting quality and mold life simultaneously.
- Necessity of the Research: Optimizing the runner system design is crucial to reduce processing time and cost in die casting. Predicting temperature distribution and residual stress at the design stage can help to balance casting quality and die life. There is a need for a robust method to model the complex relationships between die-casting parameters and performance to enable effective runner optimization.
3. Research Purpose and Research Questions:
- Research Purpose: The main purpose of this research is to design an optimized runner system for die casting dies to minimize residual stress and control temperature, thereby improving die life and casting quality. The study aims to develop a modeling approach using an abductive network and a simulation annealing algorithm to achieve this optimization.
- Key Research Questions:
- How can an abductive network be used to model the complex relationships between die-casting parameters (high-speed injector position, runner injection angle, runner sectional ratio) and die-casting performance (runner residual stress and temperature)?
- How can a simulation annealing algorithm be employed to search for optimal die-casting parameters based on the abductive network model and a defined performance index?
- How effective is the proposed runner optimization design method in reducing residual stress and controlling temperature in a die-casting die?
- Research Hypotheses:
- An abductive network can accurately predict die-casting performance based on input parameters.
- A simulation annealing algorithm can effectively identify optimal die-casting parameters that minimize residual stress and control temperature.
- Optimized runner designs, derived using the proposed method, will lead to reduced residual stress and improved die life compared to traditional designs.
4. Research Methodology
- Research Design: The research employs an experimental design approach combined with computational modeling. Runner and cavity blocks were designed and manufactured separately to allow for testing under different die-casting conditions. Twenty-three different runner insert blocks were tested.
- Data Collection Method: Die-casting experiments were conducted using an aluminum alloy. Die-casting parameters such as casting pressure (117.6 MPa), injection velocities (0.2 m/s and 2.8 m/s), and cycling time (3 shots/min) were controlled. Temperature was measured using thermocouples at points under the die cavity surface (points A-H). Residual stress was measured using the blind hole drilling method according to ASTM standards, using RS-200 drilling equipment and a SYSTEM-4000 measuring system at runner entry and near gate locations (RS in Fig. 2).
- Analysis Method: An abductive network was constructed to model the die-casting process. The network uses die-casting parameters (high-speed injector position, runner injection angle, runner sectional ratio) as inputs and predicts die-casting performance (runner residual stress and temperature). A simulation annealing algorithm was used to optimize die-casting parameters based on a performance index that combines normalized temperature and residual stress. The Predicted Square Error (PSE) criterion was used to determine the optimal network structure.
- Research Subjects and Scope: The research focuses on the runner system design in die casting dies. The experimental work involved die casting of aluminum alloy using a specifically designed die and runner blocks. The scope is limited to the optimization of runner design parameters (runner injection angle and runner sectional ratio) and high-speed injector position to minimize residual stress and control temperature near the die cavity surface.
5. Main Research Results:
- Key Research Results:
- An abductive network was successfully developed to model the die-casting process and accurately predict temperature and residual stress. The prediction errors were within 5% when compared to experimental measurements (Table 5).
- The simulation annealing algorithm effectively searched for optimal die-casting parameters.
- The residual stress at locations near the gate is approximately 100 MPa larger than at the runner entry, indicating the gate area is more prone to damage.
- The largest residual stress value was consistently found within 0.1 mm beneath the surface layer.
- Casting defects, primarily shrinkage porosity and cold shut, were observed under different experimental parameters (Table 2).
- Optimization results showed that for a runner sectional ratio of 2.3 and weight function w₁=0, w₂=1, the optimal high-speed injection position was 95.51 mm and runner injection angle was 69.1°, resulting in the lowest residual stress of 403.1 MPa (Table 6).
- For a speed injection position of 105 mm and w₁=0, w₂=1, the optimal injection angle was 72.16° and runner section ratio was 2.85, leading to a minimum residual stress of 409 MPa.
- Statistical/Qualitative Analysis Results:
- The abductive network demonstrated high prediction accuracy, with errors within 4.2% for temperature and 3.8% for residual stress.
- The simulated annealing algorithm converged to optimal parameters, demonstrating its effectiveness in optimization.
- Experimental results (Tables 3 and 4) show good agreement between measured and predicted values for temperature and residual stress.
- Data Interpretation: The results indicate that the abductive network and simulation annealing approach is a viable method for optimizing runner design in die casting. By adjusting die-casting parameters based on the optimization results, it is possible to reduce residual stress and control temperature, which are critical for enhancing die life and casting quality. The weighting function (w₁, w₂) allows for adjusting the trade-off between temperature and residual stress minimization.
- Figure Name List:
- Fig. 1. Moving cavity block.
- Fig. 2. Insert runner block.
- Fig. 3. Flow chart for the simulated annealing searching.
- Fig. 4. Effect of die-casting parameters in optimization under general conditions, when adjusting the injected position.
- Fig. 5. Effect of die-casting parameters in optimization under general conditions, when adjusting the injected angle.
- Fig. 6. Effect of die casting parameters in optimization under general condition, when adjusting the runner section ratio.
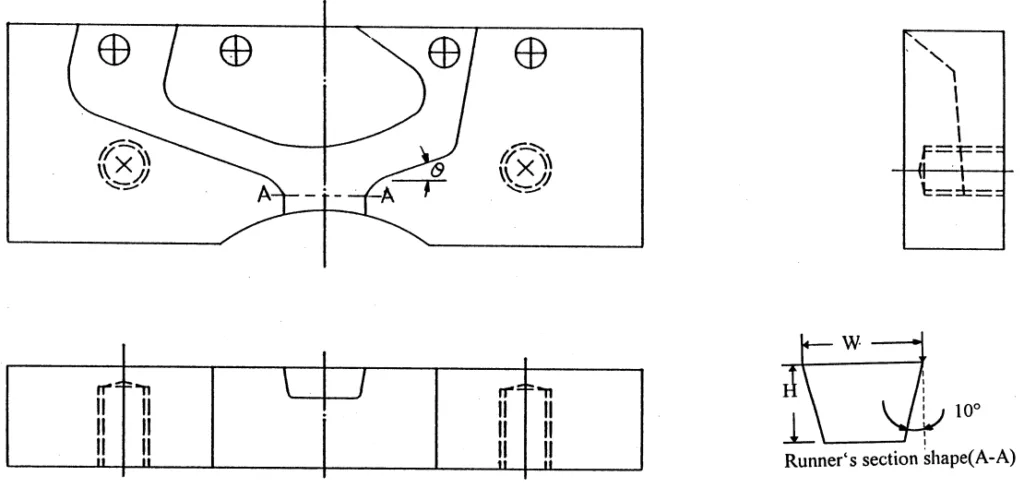
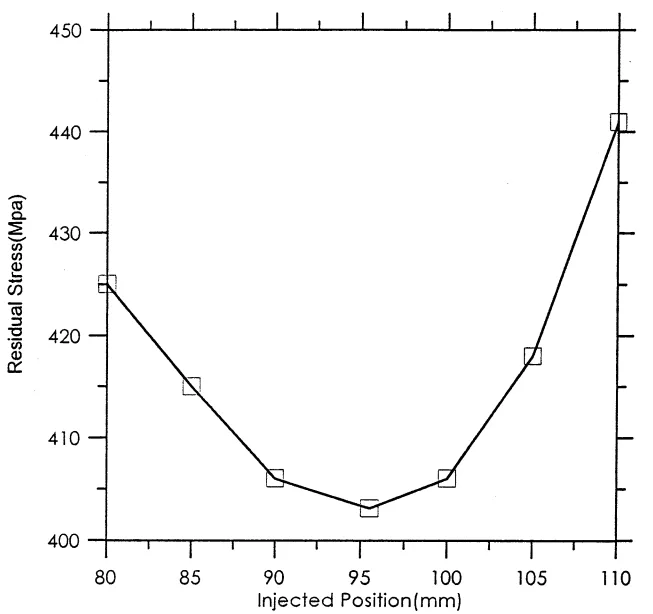
6. Conclusion and Discussion:
- Summary of Main Results: This study successfully developed and validated a runner optimization design method for die casting dies using an abductive network and simulation annealing algorithm. The method effectively models the die-casting process, predicts temperature and residual stress, and identifies optimal die-casting parameters to minimize residual stress and control temperature. Experimental validation confirmed the accuracy of the model and the effectiveness of the optimization approach.
- Academic Significance of the Research: The research contributes to the field of die casting by providing a novel approach for runner system design optimization. The application of abductive networks and simulation annealing in this context demonstrates a powerful methodology for modeling and optimizing complex manufacturing processes. The study also highlights the importance of considering both temperature and residual stress in die design for improved die life and casting quality.
- Practical Implications: The proposed method offers a practical tool for die casters to optimize runner designs, reducing the need for costly and time-consuming trial-and-error die-casting tests. By using this method, manufacturers can design dies with longer life, improved casting quality, and reduced production costs. The use of insert-style runner blocks, as demonstrated, allows for easy and cost-effective modification and optimization of runner systems.
- Limitations of the Research: The study is limited to specific die-casting parameters and materials. Further research is needed to validate the method across a broader range of die-casting conditions, materials, and die geometries. The complexity of the abductive network model and the computational cost of the simulation annealing algorithm could be considered limitations for real-time applications or very complex die designs.
7. Future Follow-up Research:
- Directions for Follow-up Research:
- Extend the application of the proposed method to different die casting alloys and die geometries.
- Investigate the influence of other die-casting parameters, such as gate design and cooling system parameters, on the optimization process.
- Explore the use of more advanced optimization algorithms to improve computational efficiency and potentially achieve even better optimization results.
- Develop a user-friendly software tool based on the proposed method to facilitate its adoption in industrial settings.
- Areas Requiring Further Exploration:
- The long-term die life improvement achieved by using optimized runner designs needs to be further investigated through prolonged die-casting experiments.
- The relationship between predicted residual stress and actual die failure mechanisms (e.g., heat checking, cracking) should be explored in more detail.
- The sensitivity of the optimization results to the weighting factors (w₁, w₂) in the objective function warrants further investigation to provide guidelines for their selection in different application scenarios.
8. References:
- [1] T.P. Groeneveld, W.D. Kaiser, Effects of metal velocity and die temperature on metal-flow distance and casting quality, Die Casting Eng. 23 (5) (1979) 44-49.
- [2] R.L. Trueloved, Die casting temperature control: a new locating waterlines, Die Casting Eng. 26 (1) (1979) 28-31.
- [3] S.H. Jong, H.Y. Chou, C.R.Li and W.S. Hwang,@ Application of mold filling analysis in the design of die casting die, Chukung Q. 73 (1992) 1-9.
- [4] S.H. Jong, M.S. Poon, H.Y. Chouand and W.S.Hwang,@ The application of solidification analysis in die casting, Chukung Q. 74 (1992) 1-11.
- [5] M. Hihara (1992) paper in Japanese.
- [6] G.J. Montgomery, K.C. Drake, Abductive reasoning network, Neurocomputing 2 (1991) 97-104.
- [7] S. Kirkpartick, C.D. Gelatt, M.P. Vecchi, Optimization by simulated annealing, Science 220 (4958) (1983) 671-680.
- [8] S. Geman, D. Geman, Stochastic relaxation, Gibbs distributions and the Bayesian restoration of images, IEEE Trans. Pattern Anal. Mach. Intell. 6 (6) (1984) 721-741.
- [9] B.W. Lee, B.J. Sheu, Hardware Annealing in Analog VLSI Neurocomputing, Kluwer, Dordrecht, 1991.
- [10] C. Zhang, H.P. Wang, The discrete tolerance optimal problem, ASME Manuf. Rev. 6 (1) (1991) 60–71.
- [11] Y.S. Trang, S.C. Ma, L.K. Chung, Determination of optimal cutting parameters in wire electrical discharge machining, Int. J. Mach. Tools Manuf. 35 (12) (1995) 1693-1701.
- [12] G.A. Miller, The magic number seven, plus or minus two: some limits on our capacity for processing information, Philos. Rev. 63 (8) (1956) 81-87.
- [13] A.R. Barron, in: S.J. Farlow (Ed.), Predicted Square Error: A Criterion for Automatic Model Selection, Self-Organizing Methods in Modeling: GMDH Type Algorithms, Marcel-Dekker, New York, 1984.
- [14] N. Metropolis, A. Rosenbluth, M. Rosenbluth, A. Teller, E. Teller, Equation of state calculation by fast computing machines, J. Chem. Phys. 21 (1953) 187-1092.
9. Copyright:
- This material is "C.C Tai, J.C Lin"'s paper: Based on "A runner-optimization design study of a die-casting die".
- Paper Source: https://doi.org/10.1016/S0924-0136(98)00031-4
This material was summarized based on the above paper, and unauthorized use for commercial purposes is prohibited.
Copyright © 2025 CASTMAN. All rights reserved.